Introducing the new Shapelet Extension
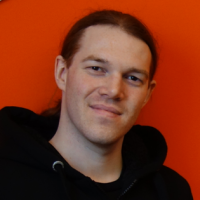

Introducing the new Shapelet Extension
We, the research department of RapidMiner, are happy to announce the release of version 0.1.0 of the new Shapelet Extension. Discover new possibilities to analyse complex time series data. Perform feature transformations of your data which are specific to your problem and are based on the underlying patterns in the time series.
The Shapelet algorithm was developed within the European Union-funded research project PRESED (see [1] and [2]), which focused on quality prediction of sensor data mining in the steel industry.
The basic idea of shapelets is that subsequences in a time series can represent a reoccurring pattern in the entire time series, and hence can be considered a base function of the time series data. In addition, some subsequences may only occur in certain classes of your data, and their occurrence can be used to train a machine learning model to predict these classes.
Image 1: Principle of Shapelet algorithm (also called EAST). [3]
To retrieve good shapelet candidates, subsequences are randomly drawn from a collection of time series batches. These candidates are then used to perform a feature transformation on a separate time series. The shapelet is then compared with a new time series batch and the minimal distance of the Shapelet candidate and the new time series batch is calculated. If the minimal distances for many batches are small, the shapelet can be considered to occur often in the time series and can then be represented as a base function.
The extension provides 4 new operators:
Create Searchspace operator:
This operator is used to draw the shapelet candidates from a collection of input batches. The candidates are collected in the new shapelet model which is provided at the 'shapelet model' result port.
Image 2: Demo process to create a shapelet model with the Create Searchspace operator.
Image 3: Visualization tab of a Shapelet Model.
Shapelet Transformation operator:
This operator takes a shapelet model and performs a feature transformation on a collection of input batches. The resulting features (e.g. the minimal distances between shapelets and the new time series) are provided at the 'features' output port.
Image 4: Demo process to perform a feature transformation with the Shapelet Transformation operator.
Image 5: Resulting feature vector of the shapelet transformation.
Select Shapelets by Weight operator:
This operator can be used to select the most meaningful shapelets from the whole shapelet model. First use the Create Searchspace operator to create a shapelet model. Then perform a shapelet transformation on labeled data and use any 'Weight by' operator to determine the weights of the calculated features according to the label. Then you can use the Attribute Weights in the Select Shapelet by Weight operator to select only the most important shapelets (base function) and apply them on unseen data
Image 6: Demo process to reduce the number of shapelets in the model to only the main shapelets, by using the Select Shapelets by Weight operator.
Image 7: Feature weights of the calculated features from the shapelet transformation.
Image 8: Reduced shapelet model, with only high feature weight shapelets selected.
Shapelet Model to ExampleSet operator
This operator can be used to convert the shapelets in a shapelet model to an ExampleSet to investigate them further.
You can download the free extension over the Marketplace (Shapelet Extension). For more information see [3]
[1] D. Arnu, E. Yaqub, C. Mocci, V. Colla, M. Neuer, G. Fricout, X. Renard, C. Mozzati and P. Gallinari: A Reference Architecture for Quality Improvement in Steel Production. 1st International Data Science Conference 2017, Salzburg
[2] D. Arnu, E. Yaqub, F. Temme, R. Klinkenberg, M. Neuer; Smart Data für die Qualitätskontrolle in der Stahlproduktion; Tagungsband 20. IFF-Wissenschaftstage; 21-22. June 2017, Magdeburg
[3] X. Renard, M. Rifqi, G. Fricout, M. Detyniecki : EAST representation: fast discovery of discriminant temporal patterns from time series, ECML/PKDD Workshop on Advanced Analytics and Learning on Temporal Data, Riva Del Garda, Italy (2016)
Comments
There should also be some communication about relevant articles posted on the net. For example, if I didn't follow Martin Schmitz @mschmitz I would have missed all his articles posted on medium. Users should not have to come across this by accident.
I have attached the relevant pdf. I hope it is possible as it would be very helpful.
regards,
Alex
Dortmund, Germany
Alex
Scott
Lexiang Ye, Eamonn J. Keogh: Time series shapelets: a new primitive for data mining. KDD 2009: 947-956
David