The Altair Community is migrating to a new platform to provide a better experience for you. In preparation for the migration, the Altair Community is on read-only mode from October 28 - November 6, 2024. Technical support via cases will continue to work as is. For any urgent requests from Students/Faculty members, please submit the form linked here
"How do I see actual vs. predicted from SVM Cross-Validation Setup?"
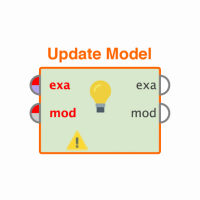

I have an SVM cross-validation setup and I want to compare actual vs. predicted. Here's my setup:
How do I get an example table of training data + predictions so that I can view / plot?
<?xml version="1.0" encoding="UTF-8" standalone="no"?>
<process version="5.1.001">
<context>
<input/>
<output/>
<macros/>
</context>
<operator activated="true" class="process" compatibility="5.1.001" expanded="true" name="Process">
<parameter key="parallelize_main_process" value="true"/>
<process expanded="true" height="615" width="964">
<operator activated="true" class="retrieve" compatibility="5.1.001" expanded="true" height="60" name="Retrieve (2)" width="90" x="179" y="30">
<parameter key="repository_entry" value="//MLData/FirstData"/>
</operator>
<operator activated="true" class="set_role" compatibility="5.1.001" expanded="true" height="76" name="Set Role (2)" width="90" x="313" y="30">
<parameter key="name" value="RRVALC"/>
<parameter key="target_role" value="weight"/>
<list key="set_additional_roles">
<parameter key="RRVAL" value="label"/>
<parameter key="Date" value="weight"/>
</list>
</operator>
<operator activated="true" class="normalize" compatibility="5.1.001" expanded="true" height="94" name="Normalize" width="90" x="447" y="30"/>
<operator activated="true" class="parallel:optimize_parameters_evolutionary_parallel" compatibility="5.0.001" expanded="true" height="112" name="Optimize Parameters (Evolutionary)" width="90" x="581" y="30">
<list key="parameters">
<parameter key="SVM.gamma" value="[0.0;10]"/>
<parameter key="SVM.nu" value="[0.0;0.5]"/>
<parameter key="SVM.C" value="[0.1;1000]"/>
<parameter key="SVM.epsilon" value="[0;15]"/>
</list>
<parameter key="max_generations" value="100"/>
<parameter key="use_early_stopping" value="true"/>
<parameter key="population_size" value="150"/>
<parameter key="show_convergence_plot" value="true"/>
<parameter key="number_of_threads" value="4"/>
<process expanded="true" height="633" width="982">
<operator activated="true" class="x_validation" compatibility="5.1.001" expanded="true" height="112" name="Validation" width="90" x="179" y="30">
<parameter key="sampling_type" value="linear sampling"/>
<process expanded="true" height="633" width="466">
<operator activated="true" class="support_vector_machine_libsvm" compatibility="5.1.001" expanded="true" height="76" name="SVM" width="90" x="179" y="30">
<parameter key="svm_type" value="epsilon-SVR"/>
<parameter key="kernel_type" value="linear"/>
<parameter key="gamma" value="0.6941252541880046"/>
<parameter key="C" value="781.8619281790575"/>
<parameter key="nu" value="0.15322895785780577"/>
<parameter key="epsilon" value="0.9220968604674066"/>
<list key="class_weights"/>
<parameter key="calculate_confidences" value="true"/>
</operator>
<connect from_port="training" to_op="SVM" to_port="training set"/>
<connect from_op="SVM" from_port="model" to_port="model"/>
<portSpacing port="source_training" spacing="0"/>
<portSpacing port="sink_model" spacing="0"/>
<portSpacing port="sink_through 1" spacing="0"/>
</process>
<process expanded="true" height="633" width="466">
<operator activated="true" class="apply_model" compatibility="5.1.001" expanded="true" height="76" name="Apply Model" width="90" x="45" y="30">
<list key="application_parameters"/>
</operator>
<operator activated="true" class="performance_regression" compatibility="5.1.001" expanded="true" height="76" name="Performance" width="90" x="246" y="30">
<parameter key="absolute_error" value="true"/>
<parameter key="relative_error" value="true"/>
</operator>
<connect from_port="model" to_op="Apply Model" to_port="model"/>
<connect from_port="test set" to_op="Apply Model" to_port="unlabelled data"/>
<connect from_op="Apply Model" from_port="labelled data" to_op="Performance" to_port="labelled data"/>
<connect from_op="Performance" from_port="performance" to_port="averagable 1"/>
<portSpacing port="source_model" spacing="0"/>
<portSpacing port="source_test set" spacing="0"/>
<portSpacing port="source_through 1" spacing="0"/>
<portSpacing port="sink_averagable 1" spacing="0"/>
<portSpacing port="sink_averagable 2" spacing="0"/>
</process>
</operator>
<connect from_port="input 1" to_op="Validation" to_port="training"/>
<connect from_op="Validation" from_port="averagable 1" to_port="performance"/>
<portSpacing port="source_input 1" spacing="0"/>
<portSpacing port="source_input 2" spacing="0"/>
<portSpacing port="sink_performance" spacing="0"/>
<portSpacing port="sink_result 1" spacing="0"/>
<portSpacing port="sink_result 2" spacing="0"/>
</process>
</operator>
<connect from_op="Retrieve (2)" from_port="output" to_op="Set Role (2)" to_port="example set input"/>
<connect from_op="Set Role (2)" from_port="example set output" to_op="Normalize" to_port="example set input"/>
<connect from_op="Normalize" from_port="example set output" to_op="Optimize Parameters (Evolutionary)" to_port="input 1"/>
<connect from_op="Optimize Parameters (Evolutionary)" from_port="performance" to_port="result 1"/>
<connect from_op="Optimize Parameters (Evolutionary)" from_port="result 1" to_port="result 2"/>
<portSpacing port="source_input 1" spacing="0"/>
<portSpacing port="sink_result 1" spacing="0"/>
<portSpacing port="sink_result 2" spacing="0"/>
<portSpacing port="sink_result 3" spacing="0"/>
</process>
</operator>
</process>
Tagged:
0
Answers
if you want to get the predictions out of a X-Validation you can replace X-Validation operator with a X-Prediction operator with the same setup.
Just add X-prediction a SVM inside after your Parameter Optimizer, that uses the optimal parameter set. You can apply the calculated optimal parameter set like described in your other thread http://rapid-i.com/rapidforum/index.php/topic,3010.msg11922.html#msg11922
The X-Prediction has a "lab" port which deliveres the labeled data.
Ciao Sebastian
Also, how do I save the best, optimal model from the X-Validation / X-Prediction run? There's no output port for model from validation operator.
this certainly is one of the most often answered questions in this forum (and even more in the old one at SourceForge a long time ago...). Fortunately I took some time to answer it in more detail one of the last times somebody asked the famous "What's the best model of my cross validation?" question. So here we go with a short link only today:
http://rapid-i.com/rapidforum/index.php/topic,62.msg1264.html
Hope that helps,
Ingo
Thanks for your response. I may not have had the right understanding of how cross-validation is used. I thought that CV simply trains a model based on part of the data and then tests the other part of data with the model in order to assess accuracy. So I was thinking that the cross validation operator would have a port to return the model if desired. What I want to see are the predicted vs. actual data for the test example set (actually, I'd be interested in seeing the entire data set too) where the predicted values are predicted from an SVM trained using the parameters found by optimal prediction operator. From Sebastian's post, I think the XML below is how one sets it up. Can someone tell me if this looks right: Ingo, I apologize if I asked the question without doing enough research. I don't mean to come here and mooch off people without giving anything back. So how about this. I made a join operator that takes multiple example sets and joins the data based on the common column ID (column ID must match, be ordered the same across all example sets, and each table must be of the same size) so the usefulness of this operator is more restricted than the general join operator, but if you think this would be a useful operator to contribute (not covered by existing operators), then I'd be happy to share my code. Let me know what you think.