The Altair Community is migrating to a new platform to provide a better experience for you. In preparation for the migration, the Altair Community is on read-only mode from October 28 - November 6, 2024. Technical support via cases will continue to work as is. For any urgent requests from Students/Faculty members, please submit the form linked here
Implementing majority votes
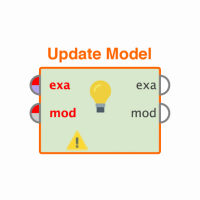

hello
I want to learn a bunch of classifiers that each work on a random subset of features and then make them vote. it is quite like a bagging feature approach.
i wrote a process that learns all these classifiers on a training set, and that computes the predictions of all classifier on the test set.
Next i want to use this collection of every classifier outputs to make them vote and decide the class of each test sample.
Yet the result of this loop is a collection of predictions, one per classifier, on the test set. This is a IOO collection with as many object as there are classifiers. The object for classifier i is an exampleset with one atribute which is the decision of the classifier on the test set.
I suppose that to compute majority vote decisions i should first transform the IOO collection in an exampleset with as manuy attributes as there are classifiers.
But I get two problems. Firsrt is transforming this IOO in an example set on which i excpect i compute majority voting decision. Second, assuming i a can transform the IOO in an example set, how to compute majority vote decisions ?
Some body could help ?
here is the code. note that it could be any dataset with real valued attributes.
I want to learn a bunch of classifiers that each work on a random subset of features and then make them vote. it is quite like a bagging feature approach.
i wrote a process that learns all these classifiers on a training set, and that computes the predictions of all classifier on the test set.
Next i want to use this collection of every classifier outputs to make them vote and decide the class of each test sample.
Yet the result of this loop is a collection of predictions, one per classifier, on the test set. This is a IOO collection with as many object as there are classifiers. The object for classifier i is an exampleset with one atribute which is the decision of the classifier on the test set.
I suppose that to compute majority vote decisions i should first transform the IOO collection in an exampleset with as manuy attributes as there are classifiers.
But I get two problems. Firsrt is transforming this IOO in an example set on which i excpect i compute majority voting decision. Second, assuming i a can transform the IOO in an example set, how to compute majority vote decisions ?
Some body could help ?
here is the code. note that it could be any dataset with real valued attributes.
<?xml version="1.0" encoding="UTF-8" standalone="no"?>
<process version="5.2.008">
<context>
<input/>
<output/>
<macros/>
</context>
<operator activated="true" class="process" compatibility="5.2.008" expanded="true" name="Process">
<process expanded="true" height="611" width="882">
<operator activated="true" class="retrieve" compatibility="5.2.008" expanded="true" height="60" name="Retrieve (2)" width="90" x="45" y="300">
<parameter key="repository_entry" value="../Datas/Datas_PAN_2012"/>
</operator>
<operator activated="true" class="normalize" compatibility="5.2.008" expanded="true" height="94" name="Normalize" width="90" x="45" y="165"/>
<operator activated="true" class="shuffle" compatibility="5.2.008" expanded="true" height="76" name="Shuffle" width="90" x="45" y="30">
<parameter key="use_local_random_seed" value="true"/>
</operator>
<operator activated="true" class="multiply" compatibility="5.2.008" expanded="true" height="94" name="Multiply" width="90" x="179" y="30"/>
<operator activated="true" class="loop" compatibility="5.2.008" expanded="true" height="94" name="Loop" width="90" x="380" y="75">
<parameter key="set_iteration_macro" value="true"/>
<parameter key="iterations" value="2"/>
<process expanded="true" height="585" width="835">
<operator activated="true" class="select_by_random" compatibility="5.2.008" expanded="true" height="76" name="Select by Random (2)" width="90" x="45" y="30">
<parameter key="use_fixed_number_of_attributes" value="true"/>
<parameter key="number_of_attributes" value="300"/>
</operator>
<operator activated="true" class="split_data" compatibility="5.2.008" expanded="true" height="94" name="Split Data (3)" width="90" x="179" y="30">
<enumeration key="partitions">
<parameter key="ratio" value="0.7"/>
<parameter key="ratio" value="0.3"/>
</enumeration>
<parameter key="sampling_type" value="linear sampling"/>
</operator>
<operator activated="true" class="support_vector_machine_libsvm" compatibility="5.2.008" expanded="true" height="76" name="SVM (3)" width="90" x="313" y="30">
<list key="class_weights"/>
</operator>
<operator activated="true" class="apply_model" compatibility="5.2.008" expanded="true" height="76" name="Apply Model (3)" width="90" x="246" y="210">
<list key="application_parameters"/>
</operator>
<operator activated="true" class="multiply" compatibility="5.2.008" expanded="true" height="94" name="Multiply (2)" width="90" x="380" y="210"/>
<operator activated="true" class="select_attributes" compatibility="5.2.008" expanded="true" height="76" name="Select Attributes (2)" width="90" x="581" y="255">
<parameter key="attribute_filter_type" value="single"/>
<parameter key="attribute" value="prediction(gensym2998)"/>
<parameter key="include_special_attributes" value="true"/>
</operator>
<operator activated="true" class="rename" compatibility="5.2.008" expanded="true" height="76" name="Rename" width="90" x="715" y="210">
<parameter key="old_name" value="prediction(gensym2998)"/>
<parameter key="new_name" value="prediction_%{iteration}"/>
<list key="rename_additional_attributes"/>
</operator>
<operator activated="true" class="performance_classification" compatibility="5.2.008" expanded="true" height="76" name="Performance (3)" width="90" x="648" y="30">
<list key="class_weights"/>
</operator>
<connect from_port="input 1" to_op="Select by Random (2)" to_port="example set input"/>
<connect from_op="Select by Random (2)" from_port="example set output" to_op="Split Data (3)" to_port="example set"/>
<connect from_op="Split Data (3)" from_port="partition 1" to_op="SVM (3)" to_port="training set"/>
<connect from_op="Split Data (3)" from_port="partition 2" to_op="Apply Model (3)" to_port="unlabelled data"/>
<connect from_op="SVM (3)" from_port="model" to_op="Apply Model (3)" to_port="model"/>
<connect from_op="Apply Model (3)" from_port="labelled data" to_op="Multiply (2)" to_port="input"/>
<connect from_op="Multiply (2)" from_port="output 1" to_op="Performance (3)" to_port="labelled data"/>
<connect from_op="Multiply (2)" from_port="output 2" to_op="Select Attributes (2)" to_port="example set input"/>
<connect from_op="Select Attributes (2)" from_port="example set output" to_op="Rename" to_port="example set input"/>
<connect from_op="Rename" from_port="example set output" to_port="output 2"/>
<connect from_op="Performance (3)" from_port="performance" to_port="output 1"/>
<portSpacing port="source_input 1" spacing="0"/>
<portSpacing port="source_input 2" spacing="0"/>
<portSpacing port="sink_output 1" spacing="0"/>
<portSpacing port="sink_output 2" spacing="0"/>
<portSpacing port="sink_output 3" spacing="0"/>
</process>
</operator>
<connect from_op="Retrieve (2)" from_port="output" to_op="Normalize" to_port="example set input"/>
<connect from_op="Normalize" from_port="example set output" to_op="Shuffle" to_port="example set input"/>
<connect from_op="Shuffle" from_port="example set output" to_op="Multiply" to_port="input"/>
<connect from_op="Multiply" from_port="output 1" to_port="result 1"/>
<connect from_op="Multiply" from_port="output 2" to_op="Loop" to_port="input 1"/>
<connect from_op="Loop" from_port="output 1" to_port="result 2"/>
<connect from_op="Loop" from_port="output 2" to_port="result 3"/>
<portSpacing port="source_input 1" spacing="0"/>
<portSpacing port="sink_result 1" spacing="0"/>
<portSpacing port="sink_result 2" spacing="0"/>
<portSpacing port="sink_result 3" spacing="0"/>
<portSpacing port="sink_result 4" spacing="0"/>
</process>
</operator>
</process>
0
Answers
I admit that I did not open your process, but maybe the Vote operator could help you. The attached process demonstrates that you can create composed models which perform a majority vote. I created 3 different models on the inside, and of course you can also perform different preprocessing etc. for the creation of each model.
Best regards,
Marius