The Altair Community is migrating to a new platform to provide a better experience for you. In preparation for the migration, the Altair Community is on read-only mode from October 28 - November 6, 2024. Technical support via cases will continue to work as is. For any urgent requests from Students/Faculty members, please submit the form linked here
Parameter Optimization, gives different results?
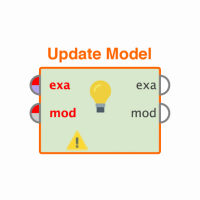

I was trying to use the grid parameter optimization to determine the optimal learning rate and momentum for a neural network classifier, I logged the AUC value as the model selection criteria, inside the parameter optimization operator I have 10-fold cross validation.
But I found out that if I run with the optimized parameter settings I got from above, just with 10-fold cross validation, the AUC value is different from the one obtained during parameter optimization. I didn't use any local random seed. The cross-validation operator is exactly the same. How could this happen?
Best regards,
But I found out that if I run with the optimized parameter settings I got from above, just with 10-fold cross validation, the AUC value is different from the one obtained during parameter optimization. I didn't use any local random seed. The cross-validation operator is exactly the same. How could this happen?
Best regards,
0
Answers
Best regards,
Marius
I used the same local random seed, but the results for AUC are still different, and not by a small amount but rather large.
The AUC obtained from parameter optimization and logged using the log operator is higher than the one I got from running x-validation with the same parameter settings by 0.05 to 0.1 which is definitely wrong.
I can replicate the results exactly the same by running the parameter optimization again they are consistent and running x-validation with the same parameter settings also have consistent results itself. It's just the two don't conincide with each other. This is really bugging me. Can anyone help and give some advice on this?
Best regards,
Marius
First, I run an experiment using parameter optimization to find the optimal learning rate for a neural network. For example, use learning rate starting from 0.1 to 1 in 10 steps, other parameters for neural network remain default. Use 10-fold stratified cross validation to get the accuracy of the classification performance. Set local random seed for both cross validation and neural network to 1992. And say for example, I get accuracy of 0.667 for learning rate 0.1 in this experiment set up.
Second, if I run the experiment without parameter optimization but only crossvalidation with neural network with learning rate 0.1 and other parameters remain default. Use the same local random seed for both cross validation and neural network. I got the accuracy of 0.6981.
I thought these two numbers should be exactly the same. But they are not and they're off quite a lot in my point of view. I don't know if the parameter optimization process is reliable or not. This also happens when I try to select the features when looping through all possible subset of features.
The two experiments are the following.
First experiment Second experiment
you are probably looking at the output of the Log operator, however that one is not configured correctly: you are logging the accuracy of the Performance operator, but that will deliver only the performance of the last iteration of the cross validation. What you want to know is the performance of the complete cross validation, so you have to log the performance value of the X-Validation.
Hope this helps!
Best regards,
Marius
For more details search the forum for 'AUC error'.
Dan
Dan