What You Don’t Know About Your Data Can Jeopardize Analysis
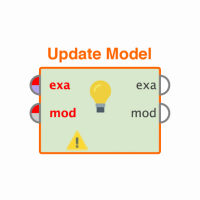

Data analyses and predictions are reliable only to the extent that the data being analyzed is understood and of acceptable quality. Building knowledge around the data and surfacing quality issues furnishes Data Scientists with the understanding necessary to construct powerful analytical models. Automated data discovery and profiling provides Data Scientists with timely visibility into enterprise data assets and their characteristics that can be leveraged to perform relevant transformations in the data pipeline, and to customize business rules to build reliable analytical models.
Data discovery and profiling products, such as Attivio Data Source Discovery, automate the process of identifying data sources and profiling the data. Data source discovery provides visibility into structured, semi-structured and unstructured data sources across an enterprise as well as those external to the enterprise. Once data sources have been gathered, each can be profiled to glean a semantic understanding of the data. This understanding encompasses data size, structure, context, quality (such as missing values or incomplete data), types of columns, statistics on columns, and relationships among the data. Profiling enables tagging and annotation, and classification of content into specific categories. The knowledge collected about the data is available to Data Scientists in the form of “metadata.”
A representative use case for illustrating the benefits of automated data discovery and profiling is that of missing, or incomplete data. Missing data is pervasive, occurring over a broad range of domains including biomedical and behavioral sciences, sociology, economics, and political science. Similarly, missing data can stem from a variety of situations including:
* Non-responsiveness by census participants to survey questions of a sensitive nature such as ethnicity, nationality, or income.
* Poorly designed surveys in which some questions may be ambiguous.
* Errors or exclusions in self-reporting by participants in behavioral and medical science studies.
* Subjects in a clinical trial may discontinue their participation before conclusion of the study, resulting in incomplete data.
* Mistakes by a researcher in collecting or entering data, resulting in incorrect or missing values.
* Incomplete sensor (telemetry) data owing to disruptions in data ingestion or failure of a sensor.
Data quality in part hinges on the extent and types of missing values occurring in the data. There is no pragmatic nor quantitative justification for perpetuating missing values. Products like Attivio Data Source Discovery enable automated identification, flagging, and tagging of missing values. The corresponding metadata is indispensable to the Data Scientist in properly handling the missing values in accordance with the specific domain being modeled for analysis. A predictive analytics platform such as RapidMiner empowers Data Scientists to cleanse and transform missing data values in advance of creating or modifying, and executing analytical models.
Enterprises clearly benefit in several ways from leveraging a data discovery and profiling product in tandem with a predictive analytics product. Automatically gathering data sources, characterizing the data, and surfacing quality issues releases the Data Scientist to concentrate efforts on his primary responsibility, that of building meaningful analytical models. The result is improved efficiency and productivity, and potentially reduction in costs. Another advantage of using the two products in combination is that metadata generated from data discovery and profiling is accessible to predictive analytics, thus facilitating data handling (cleansing and transforming) and model building by the Data Scientist on the analytics platform. If, in the future, data sources are added or removed, this will be reflected in the metadata and thus enable updates to data models that might otherwise turn “stale.”