The Altair Community is migrating to a new platform to provide a better experience for you. In preparation for the migration, the Altair Community is on read-only mode from October 28 - November 6, 2024. Technical support via cases will continue to work as is. For any urgent requests from Students/Faculty members, please submit the form linked here
"Insufficient results with M5P regression tree"
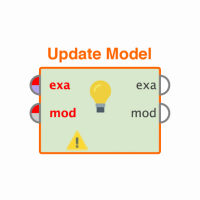

Hello,
if anyone is interested please try the following:
produce a file containing two columns
x = 0, 0.1, 0.2, ..., 12.6;
y = sin(x)
Then apply M5P (with or without normalization).
The result is quite disappointing. Does anyone know how to get an acceptable result?
I expected to get something like a picewise linear approximation of the sin function,
but got something far away from this.
Thank You.
if anyone is interested please try the following:
produce a file containing two columns
x = 0, 0.1, 0.2, ..., 12.6;
y = sin(x)
Then apply M5P (with or without normalization).
The result is quite disappointing. Does anyone know how to get an acceptable result?
I expected to get something like a picewise linear approximation of the sin function,
but got something far away from this.
Thank You.
Tagged:
0
Answers
I just tried the following process, and the only changes from the default settings are to click the check box for parameters N, U, and R : And the plot of x vs. prediction(y) looks, to my eyes, much more sin-like. But I don't know if using an unpruned, unsmoothed learner makes sense for your problem.
Keith
sorry, here is the XML
<operator name="Root" class="Process" expanded="yes">
<operator name="ExampleSource" class="ExampleSource">
<parameter key="attributes" value="C:\Programme\Rapid-I\RapidMiner-4.4\sinus"/>
</operator>
<operator name="Normalization" class="Normalization">
</operator>
<operator name="W-M5P" class="W-M5P">
<parameter key="keep_example_set" value="true"/>
<parameter key="U" value="true"/>
<parameter key="M" value="10.0"/>
</operator>
<operator name="ModelApplier" class="ModelApplier">
<list key="application_parameters">
</list>
</operator>
</operator>
What I get is a piecewise constant result, i.e. the leafes of the tree are: y = const
Only the last leaf gives a linear model: y = 3.2196 * x - 4.5545
If I had such a "really" linear model at all leafes of the tree, it would be ok, i.e. as
I would expect it.
There are no settings which can improve it, even if the tree could result in y = a*x+b
in each leaf, which should give a better prediction. So why does'nt M5P behave like
this?
If I select the smoothed tree the results are even worse.
I hope I could make my "problem" more clear to you.
P.S.:
Maybe if you google for "stepwise regression tree HUANG" or go directly to
http://www.landcover.org/pdf/ijrs24_p75.pdf
and there at page 77 (i.e. page 3 in the 16 pages document) you see what I
mean. If this SRT algorithm would become a part of RapidMiner I would
appreciate it
Nevertheless, I cannot understand, why the fraction of constant leafs, i.e. y = const, increases if I change M from 5 to 6.
I get 10 constant leafes more at positions where y = a*x+b would be better. Isn't the result with a constant regression
worse than a non constant regression in the leafs?
It's clear to me that thealgorithm is from Weka and not RapidMiner, so You cannot know in detail what happens.
Nevertheless, I only want to understand, why, by increasing M, the number of constant leafs increases even
if it worses the result.
By the way, if you are an expert
Up to now I didn't get the right feeling for applying meta methods like grid search or x-validation in the right way.
Thank's in advance. (At least I need an answer on my question, the workflow would be nice)
As for the parameter optimization, take a look at 07_Meta/01_ParameterOptimization.xml in the RM samples directory. The GridParameterOptimization node is where you'd specify what parameters you want to tinker with.
The problem where I tested M5P was originally only for me to get an idea how M5P works.
Finally I'm really in doubt applying this method to other data that I'm not familiar to.