The Altair Community is migrating to a new platform to provide a better experience for you. In preparation for the migration, the Altair Community is on read-only mode from October 28 - November 6, 2024. Technical support via cases will continue to work as is. For any urgent requests from Students/Faculty members, please submit the form linked here
Help for Missing KNN Variable
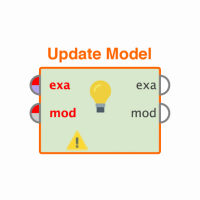

Hey guys, I uploaded the following process of mine. Everything worked well so far. Goal was to predict the variables H N U .. I get a perfect result for the variables H N but not a single U . But I know for sure that you can predict U as well. Maybe decision tree is also a good alternative?
If someone could have a look at it and come up with a solution I would be very grateful.
cheers
If someone could have a look at it and come up with a solution I would be very grateful.
cheers
<?xml version="1.0" encoding="UTF-8"?><process version="9.1.000">
<context>
<input/>
<output/>
<macros/>
</context>
<operator activated="true" class="process" compatibility="9.1.000" expanded="true" name="Process">
<parameter key="logverbosity" value="init"/>
<parameter key="random_seed" value="2001"/>
<parameter key="send_mail" value="never"/>
<parameter key="notification_email" value=""/>
<parameter key="process_duration_for_mail" value="30"/>
<parameter key="encoding" value="SYSTEM"/>
<process expanded="true">
<operator activated="true" class="retrieve" compatibility="9.1.000" expanded="true" height="68" name="Retrieve retouren_class" width="90" x="45" y="391">
<parameter key="repository_entry" value="retouren_class"/>
</operator>
<operator activated="true" class="set_role" compatibility="9.1.000" expanded="true" height="82" name="Set Role (2)" width="90" x="179" y="391">
<parameter key="attribute_name" value="KDNR"/>
<parameter key="target_role" value="id"/>
<list key="set_additional_roles"/>
</operator>
<operator activated="true" class="replace_missing_values" compatibility="9.1.000" expanded="true" height="103" name="Replace Missing Values (2)" width="90" x="380" y="391">
<parameter key="return_preprocessing_model" value="false"/>
<parameter key="create_view" value="false"/>
<parameter key="attribute_filter_type" value="all"/>
<parameter key="attribute" value=""/>
<parameter key="attributes" value=""/>
<parameter key="use_except_expression" value="false"/>
<parameter key="value_type" value="attribute_value"/>
<parameter key="use_value_type_exception" value="false"/>
<parameter key="except_value_type" value="time"/>
<parameter key="block_type" value="attribute_block"/>
<parameter key="use_block_type_exception" value="false"/>
<parameter key="except_block_type" value="value_matrix_row_start"/>
<parameter key="invert_selection" value="false"/>
<parameter key="include_special_attributes" value="false"/>
<parameter key="default" value="average"/>
<list key="columns"/>
</operator>
<operator activated="true" class="retrieve" compatibility="9.1.000" expanded="true" height="68" name="Retrieve retouren_train" width="90" x="45" y="187">
<parameter key="repository_entry" value="retouren_train"/>
</operator>
<operator activated="true" class="replace_missing_values" compatibility="9.1.000" expanded="true" height="103" name="Replace Missing Values" width="90" x="179" y="136">
<parameter key="return_preprocessing_model" value="false"/>
<parameter key="create_view" value="false"/>
<parameter key="attribute_filter_type" value="no_missing_values"/>
<parameter key="attribute" value=""/>
<parameter key="attributes" value=""/>
<parameter key="use_except_expression" value="false"/>
<parameter key="value_type" value="attribute_value"/>
<parameter key="use_value_type_exception" value="false"/>
<parameter key="except_value_type" value="time"/>
<parameter key="block_type" value="attribute_block"/>
<parameter key="use_block_type_exception" value="false"/>
<parameter key="except_block_type" value="value_matrix_row_start"/>
<parameter key="invert_selection" value="false"/>
<parameter key="include_special_attributes" value="false"/>
<parameter key="default" value="average"/>
<list key="columns"/>
</operator>
<operator activated="true" class="generate_attributes" compatibility="9.1.000" expanded="true" height="82" name="Generate Attributes" width="90" x="313" y="136">
<list key="function_descriptions">
<parameter key="Retourenquote" value="RETOUREN_MENGE/LIEFER_MENGE"/>
<parameter key="Zuordnung" value="if(Retourenquote<0.18,"N",if(Retourenquote>0.40,"H","U"))"/>
</list>
<parameter key="keep_all" value="true"/>
</operator>
<operator activated="true" class="set_role" compatibility="9.1.000" expanded="true" height="82" name="Set Role" width="90" x="447" y="136">
<parameter key="attribute_name" value="KDNR"/>
<parameter key="target_role" value="id"/>
<list key="set_additional_roles">
<parameter key="Zuordnung" value="label"/>
</list>
</operator>
<operator activated="true" class="select_attributes" compatibility="9.1.000" expanded="true" height="82" name="Select Attributes" width="90" x="514" y="238">
<parameter key="attribute_filter_type" value="subset"/>
<parameter key="attribute" value=""/>
<parameter key="attributes" value="AADSEIT|AATELKZ|APLZ5ST|APLZAAH|APLZAKH|APLZBVD|APLZEIN|APLZFLH|APLZFLI|APLZKKE|BBH0001|BBH0002|BBW0001|BBW0002|BBW0003|BEB0001|BLB0001|BRP0001|BRP0002|BRP0003|BRP0004|BRQ0001|BRQ0002|BRQ0003|BRQ0004|BRW0001|LRQ0001|LRQ0002|LRQ0003|LRQ0004|PALTERK|PANREDK|PRSANZW|WRQ0001|WRQ0002|WRQ0003|WRQ0004|WRQ0005|WRQ0006|WRQ0007|WRQ0008|WRQ0009|WRQ0010|WRQ0011|WRQ0012|WRQ0013|WRQ0014|WRQ0015|WRQ0016|WRW0001|WRW0002|WRW0003|WRW0004|WRW0005|WRW0006|WRW0007|WRW0008|WRW0009|WRW0010|WRW0011|WRW0012|WRW0013|WRW0014|WRW0015|WRW0016|KDNR"/>
<parameter key="use_except_expression" value="false"/>
<parameter key="value_type" value="attribute_value"/>
<parameter key="use_value_type_exception" value="false"/>
<parameter key="except_value_type" value="time"/>
<parameter key="block_type" value="attribute_block"/>
<parameter key="use_block_type_exception" value="false"/>
<parameter key="except_block_type" value="value_matrix_row_start"/>
<parameter key="invert_selection" value="false"/>
<parameter key="include_special_attributes" value="false"/>
</operator>
<operator activated="true" class="concurrency:optimize_parameters_grid" compatibility="9.1.000" expanded="true" height="124" name="Optimize Parameters (Grid)" width="90" x="700" y="136">
<list key="parameters">
<parameter key="k-NN.k" value="[1.0;100.0;10;linear]"/>
</list>
<parameter key="error_handling" value="fail on error"/>
<parameter key="log_performance" value="true"/>
<parameter key="log_all_criteria" value="false"/>
<parameter key="synchronize" value="false"/>
<parameter key="enable_parallel_execution" value="true"/>
<process expanded="true">
<operator activated="true" class="concurrency:cross_validation" compatibility="9.1.000" expanded="true" height="145" name="Cross Validation" width="90" x="112" y="187">
<parameter key="split_on_batch_attribute" value="false"/>
<parameter key="leave_one_out" value="false"/>
<parameter key="number_of_folds" value="10"/>
<parameter key="sampling_type" value="automatic"/>
<parameter key="use_local_random_seed" value="false"/>
<parameter key="local_random_seed" value="1992"/>
<parameter key="enable_parallel_execution" value="true"/>
<process expanded="true">
<operator activated="true" class="normalize" compatibility="9.1.000" expanded="true" height="103" name="Normalize" width="90" x="45" y="238">
<parameter key="return_preprocessing_model" value="false"/>
<parameter key="create_view" value="false"/>
<parameter key="attribute_filter_type" value="all"/>
<parameter key="attribute" value=""/>
<parameter key="attributes" value=""/>
<parameter key="use_except_expression" value="false"/>
<parameter key="value_type" value="numeric"/>
<parameter key="use_value_type_exception" value="false"/>
<parameter key="except_value_type" value="real"/>
<parameter key="block_type" value="value_series"/>
<parameter key="use_block_type_exception" value="false"/>
<parameter key="except_block_type" value="value_series_end"/>
<parameter key="invert_selection" value="false"/>
<parameter key="include_special_attributes" value="false"/>
<parameter key="method" value="Z-transformation"/>
<parameter key="min" value="0.0"/>
<parameter key="max" value="1.0"/>
<parameter key="allow_negative_values" value="false"/>
</operator>
<operator activated="true" class="k_nn" compatibility="9.1.000" expanded="true" height="82" name="k-NN" width="90" x="246" y="238">
<parameter key="k" value="100"/>
<parameter key="weighted_vote" value="true"/>
<parameter key="measure_types" value="MixedMeasures"/>
<parameter key="mixed_measure" value="MixedEuclideanDistance"/>
<parameter key="nominal_measure" value="NominalDistance"/>
<parameter key="numerical_measure" value="EuclideanDistance"/>
<parameter key="divergence" value="GeneralizedIDivergence"/>
<parameter key="kernel_type" value="radial"/>
<parameter key="kernel_gamma" value="1.0"/>
<parameter key="kernel_sigma1" value="1.0"/>
<parameter key="kernel_sigma2" value="0.0"/>
<parameter key="kernel_sigma3" value="2.0"/>
<parameter key="kernel_degree" value="3.0"/>
<parameter key="kernel_shift" value="1.0"/>
<parameter key="kernel_a" value="1.0"/>
<parameter key="kernel_b" value="0.0"/>
</operator>
<operator activated="true" class="group_models" compatibility="9.1.000" expanded="true" height="103" name="Group Models" width="90" x="139" y="34"/>
<connect from_port="training set" to_op="Normalize" to_port="example set input"/>
<connect from_op="Normalize" from_port="example set output" to_op="k-NN" to_port="training set"/>
<connect from_op="Normalize" from_port="preprocessing model" to_op="Group Models" to_port="models in 1"/>
<connect from_op="k-NN" from_port="model" to_op="Group Models" to_port="models in 2"/>
<connect from_op="Group Models" from_port="model out" to_port="model"/>
<portSpacing port="source_training set" spacing="0"/>
<portSpacing port="sink_model" spacing="0"/>
<portSpacing port="sink_through 1" spacing="0"/>
</process>
<process expanded="true">
<operator activated="true" class="apply_model" compatibility="9.1.000" expanded="true" height="82" name="Apply Model (2)" width="90" x="45" y="85">
<list key="application_parameters"/>
<parameter key="create_view" value="false"/>
</operator>
<operator activated="true" class="performance_classification" compatibility="9.1.000" expanded="true" height="82" name="Performance" width="90" x="112" y="238">
<parameter key="main_criterion" value="first"/>
<parameter key="accuracy" value="true"/>
<parameter key="classification_error" value="false"/>
<parameter key="kappa" value="false"/>
<parameter key="weighted_mean_recall" value="false"/>
<parameter key="weighted_mean_precision" value="false"/>
<parameter key="spearman_rho" value="false"/>
<parameter key="kendall_tau" value="false"/>
<parameter key="absolute_error" value="false"/>
<parameter key="relative_error" value="false"/>
<parameter key="relative_error_lenient" value="false"/>
<parameter key="relative_error_strict" value="false"/>
<parameter key="normalized_absolute_error" value="false"/>
<parameter key="root_mean_squared_error" value="false"/>
<parameter key="root_relative_squared_error" value="false"/>
<parameter key="squared_error" value="false"/>
<parameter key="correlation" value="false"/>
<parameter key="squared_correlation" value="false"/>
<parameter key="cross-entropy" value="false"/>
<parameter key="margin" value="false"/>
<parameter key="soft_margin_loss" value="false"/>
<parameter key="logistic_loss" value="false"/>
<parameter key="skip_undefined_labels" value="true"/>
<parameter key="use_example_weights" value="true"/>
<list key="class_weights"/>
</operator>
<connect from_port="model" to_op="Apply Model (2)" to_port="model"/>
<connect from_port="test set" to_op="Apply Model (2)" to_port="unlabelled data"/>
<connect from_op="Apply Model (2)" from_port="labelled data" to_op="Performance" to_port="labelled data"/>
<connect from_op="Performance" from_port="performance" to_port="performance 1"/>
<portSpacing port="source_model" spacing="0"/>
<portSpacing port="source_test set" spacing="0"/>
<portSpacing port="source_through 1" spacing="0"/>
<portSpacing port="sink_test set results" spacing="0"/>
<portSpacing port="sink_performance 1" spacing="0"/>
<portSpacing port="sink_performance 2" spacing="0"/>
</process>
</operator>
<connect from_port="input 1" to_op="Cross Validation" to_port="example set"/>
<connect from_op="Cross Validation" from_port="model" to_port="model"/>
<connect from_op="Cross Validation" from_port="performance 1" to_port="performance"/>
<portSpacing port="source_input 1" spacing="0"/>
<portSpacing port="source_input 2" spacing="0"/>
<portSpacing port="sink_performance" spacing="0"/>
<portSpacing port="sink_model" spacing="0"/>
<portSpacing port="sink_output 1" spacing="0"/>
</process>
</operator>
<operator activated="true" class="apply_model" compatibility="9.1.000" expanded="true" height="82" name="Apply Model" width="90" x="581" y="391">
<list key="application_parameters"/>
<parameter key="create_view" value="false"/>
</operator>
<operator activated="true" class="write_excel" compatibility="9.1.000" expanded="true" height="82" name="Write Excel" width="90" x="700" y="340">
<parameter key="excel_file" value="/Users/Admin1/Desktop/Du huan.xlsx"/>
<parameter key="file_format" value="xlsx"/>
<parameter key="encoding" value="SYSTEM"/>
<parameter key="sheet_name" value="RapidMiner Data"/>
<parameter key="date_format" value="yyyy-MM-dd HH:mm:ss"/>
<parameter key="number_format" value="#.0"/>
</operator>
<connect from_op="Retrieve retouren_class" from_port="output" to_op="Set Role (2)" to_port="example set input"/>
<connect from_op="Set Role (2)" from_port="example set output" to_op="Replace Missing Values (2)" to_port="example set input"/>
<connect from_op="Replace Missing Values (2)" from_port="example set output" to_op="Apply Model" to_port="unlabelled data"/>
<connect from_op="Retrieve retouren_train" from_port="output" to_op="Replace Missing Values" to_port="example set input"/>
<connect from_op="Replace Missing Values" from_port="example set output" to_op="Generate Attributes" to_port="example set input"/>
<connect from_op="Generate Attributes" from_port="example set output" to_op="Set Role" to_port="example set input"/>
<connect from_op="Set Role" from_port="example set output" to_op="Select Attributes" to_port="example set input"/>
<connect from_op="Select Attributes" from_port="example set output" to_op="Optimize Parameters (Grid)" to_port="input 1"/>
<connect from_op="Optimize Parameters (Grid)" from_port="performance" to_port="result 1"/>
<connect from_op="Optimize Parameters (Grid)" from_port="model" to_op="Apply Model" to_port="model"/>
<connect from_op="Apply Model" from_port="labelled data" to_op="Write Excel" to_port="input"/>
<connect from_op="Write Excel" from_port="through" to_port="result 2"/>
<portSpacing port="source_input 1" spacing="0"/>
<portSpacing port="sink_result 1" spacing="0"/>
<portSpacing port="sink_result 2" spacing="0"/>
<portSpacing port="sink_result 3" spacing="0"/>
</process>
</operator>
</process>
Tagged:
0
Answers
Difficult to propose a solution without running your process with your dataset(s) ==> Can you share them ?
However I checked your process. One important step in the data-science methodology is to apply the same preprocessing steps in your training
set, in your test set and in your scoreset , so in your case :
- apply Normalization to your test set inside the Cross Validation operator like that :
- Apply Normalization operator to your score set
- Apply Select Attributes operator to your score set (the attributes in your training set and in your score set must be the same)
I hope it helps,
Regards,
Lionel
You have an imbalanced dataset. Your "U" class is a minority in your training dataset :
In such cases, the algorithms have difficulties to establish correlation between this class of your target variable
and your regular attributes.
What you observe is that when you score a test test, you have no "U" as predicted class.
For example, you choose to train and optimize a kNN model based on accuracy => RM concludes to k = 41 Neighbours.
When you score a new point of a test set, the probability that your test point is surrounded by a majority of training points belonging to the "U" class
is very low and in practice, in your case, is null, that's why you have no "U" as predicted class :
If your goal is to correctly detect/predict the class "U", a solution is to train a model after sampling (increasing the proportion of your "U" class in your training dataset) your training set and set the main criterion of the Performance operator to weighted_mean_recall.(in the Cross Validation operator).
For example with these proportions :
you obtain these results :
Hope it's clearer now,
Regards,
Lionel