The Altair Community is migrating to a new platform to provide a better experience for you. In preparation for the migration, the Altair Community is on read-only mode from October 28 - November 6, 2024. Technical support via cases will continue to work as is. For any urgent requests from Students/Faculty members, please submit the form linked here
"Algorithm in Cross Validation keep on processing"
Hi,
I am trying to do cross-validation 5 fold, but the SVM and Logistic regression operators in CV keep on processing as shown in the figure below. Its been 10 hours and they are same. The dataset size is 316974 samples with 40 attributes. XML below. Is this due to dataset size? Generally, it needs to show some percentage of completion but it keeps on processing without change.
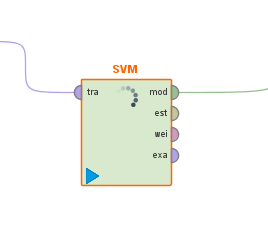
Thanks,
Varun
I am trying to do cross-validation 5 fold, but the SVM and Logistic regression operators in CV keep on processing as shown in the figure below. Its been 10 hours and they are same. The dataset size is 316974 samples with 40 attributes. XML below. Is this due to dataset size? Generally, it needs to show some percentage of completion but it keeps on processing without change.
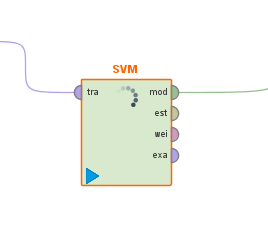
<?xml version="1.0" encoding="UTF-8"?><process version="9.1.000"> <context> <input/> <output/> <macros/> </context> <operator activated="true" class="process" compatibility="9.1.000" expanded="true" name="Process"> <parameter key="logverbosity" value="init"/> <parameter key="random_seed" value="2001"/> <parameter key="send_mail" value="never"/> <parameter key="notification_email" value=""/> <parameter key="process_duration_for_mail" value="30"/> <parameter key="encoding" value="SYSTEM"/> <process expanded="true"> <operator activated="true" class="retrieve" compatibility="9.1.000" expanded="true" height="68" name="Retrieve Assistment_Data_Feature_Selection" width="90" x="45" y="136"> <parameter key="repository_entry" value="../../data/AIED_New/Assistment_Data_Feature_Selection"/> </operator> <operator activated="true" class="select_attributes" compatibility="9.1.000" expanded="true" height="82" name="Select Attributes" width="90" x="246" y="136"> <parameter key="attribute_filter_type" value="subset"/> <parameter key="attribute" value=""/> <parameter key="attributes" value="Ln|Ln-1|NumActions|RES_BORED|RES_CONFUSED|RES_FRUSTRATED|RES_GAMING|RES_OFFTASK|Skill_ID|attemptCount|correct|endsWithScaffolding|frIsHelpRequest|frIsHelpRequestScaffolding|frPast5HelpRequest|frPast5WrongCount|frPast8HelpRequest|frPast8WrongCount|frTotalSkillOpportunitiesScaffolding|hint|hintCount|hintTotal|manywrong|original|past8BottomOut|scaffold|sumRight|sumTimePerSkill|timeGreater10SecAndNextActionRight|timeSinceSkill|timeTaken|totalFrAttempted|totalFrPastWrongCount|totalFrPercentPastWrong|totalFrSkillOpportunities|totalFrSkillOpportunitiesByScaffolding|totalFrTimeOnSkill|totalTimeByPercentCorrectForskill"/> <parameter key="use_except_expression" value="false"/> <parameter key="value_type" value="attribute_value"/> <parameter key="use_value_type_exception" value="false"/> <parameter key="except_value_type" value="time"/> <parameter key="block_type" value="attribute_block"/> <parameter key="use_block_type_exception" value="false"/> <parameter key="except_block_type" value="value_matrix_row_start"/> <parameter key="invert_selection" value="false"/> <parameter key="include_special_attributes" value="false"/> </operator> <operator activated="true" class="multiply" compatibility="9.1.000" expanded="true" height="82" name="Multiply" width="90" x="380" y="34"/> <operator activated="true" class="concurrency:cross_validation" compatibility="9.1.000" expanded="true" height="166" name="Cross Validation" width="90" x="581" y="136"> <parameter key="split_on_batch_attribute" value="false"/> <parameter key="leave_one_out" value="false"/> <parameter key="number_of_folds" value="5"/> <parameter key="sampling_type" value="automatic"/> <parameter key="use_local_random_seed" value="true"/> <parameter key="local_random_seed" value="1992"/> <parameter key="enable_parallel_execution" value="true"/> <process expanded="true"> <operator activated="true" class="support_vector_machine" compatibility="9.1.000" expanded="true" height="124" name="SVM" width="90" x="112" y="85"> <parameter key="kernel_type" value="dot"/> <parameter key="kernel_gamma" value="1.0"/> <parameter key="kernel_sigma1" value="1.0"/> <parameter key="kernel_sigma2" value="0.0"/> <parameter key="kernel_sigma3" value="2.0"/> <parameter key="kernel_shift" value="1.0"/> <parameter key="kernel_degree" value="2.0"/> <parameter key="kernel_a" value="1.0"/> <parameter key="kernel_b" value="0.0"/> <parameter key="kernel_cache" value="200"/> <parameter key="C" value="0.0"/> <parameter key="convergence_epsilon" value="0.001"/> <parameter key="max_iterations" value="100000"/> <parameter key="scale" value="true"/> <parameter key="calculate_weights" value="true"/> <parameter key="return_optimization_performance" value="true"/> <parameter key="L_pos" value="1.0"/> <parameter key="L_neg" value="1.0"/> <parameter key="epsilon" value="0.0"/> <parameter key="epsilon_plus" value="0.0"/> <parameter key="epsilon_minus" value="0.0"/> <parameter key="balance_cost" value="false"/> <parameter key="quadratic_loss_pos" value="false"/> <parameter key="quadratic_loss_neg" value="false"/> <parameter key="estimate_performance" value="false"/> </operator> <connect from_port="training set" to_op="SVM" to_port="training set"/> <connect from_op="SVM" from_port="model" to_port="model"/> <portSpacing port="source_training set" spacing="0"/> <portSpacing port="sink_model" spacing="0"/> <portSpacing port="sink_through 1" spacing="0"/> </process> <process expanded="true"> <operator activated="true" class="apply_model" compatibility="9.1.000" expanded="true" height="82" name="Apply Model" width="90" x="45" y="34"> <list key="application_parameters"/> <parameter key="create_view" value="false"/> </operator> <operator activated="true" class="multiply" compatibility="9.1.000" expanded="true" height="103" name="Multiply (2)" width="90" x="45" y="136"/> <operator activated="true" class="performance" compatibility="9.1.000" expanded="true" height="82" name="Performance" width="90" x="179" y="238"> <parameter key="use_example_weights" value="true"/> </operator> <operator activated="true" class="performance_classification" compatibility="9.1.000" expanded="true" height="82" name="Performance (2)" width="90" x="246" y="34"> <parameter key="main_criterion" value="first"/> <parameter key="accuracy" value="true"/> <parameter key="classification_error" value="false"/> <parameter key="kappa" value="true"/> <parameter key="weighted_mean_recall" value="false"/> <parameter key="weighted_mean_precision" value="false"/> <parameter key="spearman_rho" value="false"/> <parameter key="kendall_tau" value="false"/> <parameter key="absolute_error" value="false"/> <parameter key="relative_error" value="false"/> <parameter key="relative_error_lenient" value="false"/> <parameter key="relative_error_strict" value="false"/> <parameter key="normalized_absolute_error" value="false"/> <parameter key="root_mean_squared_error" value="true"/> <parameter key="root_relative_squared_error" value="false"/> <parameter key="squared_error" value="false"/> <parameter key="correlation" value="false"/> <parameter key="squared_correlation" value="false"/> <parameter key="cross-entropy" value="false"/> <parameter key="margin" value="false"/> <parameter key="soft_margin_loss" value="false"/> <parameter key="logistic_loss" value="false"/> <parameter key="skip_undefined_labels" value="true"/> <parameter key="use_example_weights" value="true"/> <list key="class_weights"/> </operator> <connect from_port="model" to_op="Apply Model" to_port="model"/> <connect from_port="test set" to_op="Apply Model" to_port="unlabelled data"/> <connect from_op="Apply Model" from_port="labelled data" to_op="Multiply (2)" to_port="input"/> <connect from_op="Multiply (2)" from_port="output 1" to_op="Performance" to_port="labelled data"/> <connect from_op="Multiply (2)" from_port="output 2" to_op="Performance (2)" to_port="labelled data"/> <connect from_op="Performance" from_port="performance" to_port="performance 2"/> <connect from_op="Performance (2)" from_port="performance" to_port="performance 1"/> <portSpacing port="source_model" spacing="0"/> <portSpacing port="source_test set" spacing="0"/> <portSpacing port="source_through 1" spacing="0"/> <portSpacing port="sink_test set results" spacing="0"/> <portSpacing port="sink_performance 1" spacing="0"/> <portSpacing port="sink_performance 2" spacing="0"/> <portSpacing port="sink_performance 3" spacing="0"/> </process> </operator> <connect from_op="Retrieve Assistment_Data_Feature_Selection" from_port="output" to_op="Select Attributes" to_port="example set input"/> <connect from_op="Select Attributes" from_port="example set output" to_op="Multiply" to_port="input"/> <connect from_op="Multiply" from_port="output 1" to_op="Cross Validation" to_port="example set"/> <connect from_op="Cross Validation" from_port="performance 1" to_port="result 1"/> <connect from_op="Cross Validation" from_port="performance 2" to_port="result 2"/> <portSpacing port="source_input 1" spacing="0"/> <portSpacing port="sink_result 1" spacing="0"/> <portSpacing port="sink_result 2" spacing="0"/> <portSpacing port="sink_result 3" spacing="0"/> </process> </operator> </process>
Varun
Regards,
Varun
https://www.varunmandalapu.com/
Varun
https://www.varunmandalapu.com/
Be Safe. Follow precautions and Maintain Social Distancing
Tagged:
0
Best Answer
-
MartinLiebig Administrator, Moderator, Employee-RapidMiner, RapidMiner Certified Analyst, RapidMiner Certified Expert, University Professor Posts: 3,533
RM Data Scientist
Hi @varunm1 ,300k is simply a lot for an SVM. SVMs scale well in #attributes, but not so well in #samples.
Sadly there is one librarly which does not offer a kill-switch. If i remember correctly RM cannot kill it from the outside and you would need to kill the java process.BR,Martin- Sr. Director Data Solutions, Altair RapidMiner -
Dortmund, Germany6
Answers
Varun
https://www.varunmandalapu.com/
Be Safe. Follow precautions and Maintain Social Distancing
Lindon Ventures
Data Science Consulting from Certified RapidMiner Experts
Varun
https://www.varunmandalapu.com/
Be Safe. Follow precautions and Maintain Social Distancing
Dortmund, Germany