The Altair Community is migrating to a new platform to provide a better experience for you. In preparation for the migration, the Altair Community is on read-only mode from October 28 - November 6, 2024. Technical support via cases will continue to work as is. For any urgent requests from Students/Faculty members, please submit the form linked here
Validation Performance Issue
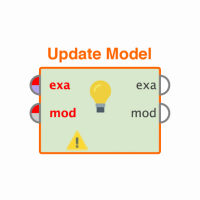

Hi everyone,
I have a question, when i apply both cross validation and split validation at one time using multiply, the performance results of either cross validation operator or split validation operator have difference accuracy with when i only apply one of cross validation or split validation separately ( i mean i enable one of them ), why ? I provide both two scripts one for when apply both and one of only cross validation
*** This one script for process when apply both cross val and split val
Thank you
*** This one script for process when apply both cross val and split val
<?xml version="1.0" encoding="UTF-8"?><process version="9.3.001"> <operator activated="true" class="retrieve" compatibility="9.3.001" expanded="true" height="68" name="Retrieve Customer Data" origin="GENERATED_SAMPLE" width="90" x="45" y="136"> <parameter key="repository_entry" value="//Samples/Templates/Churn Modeling/Customer Data"/> </operator> </process> <?xml version="1.0" encoding="UTF-8"?><process version="9.3.001"> <operator activated="true" class="set_role" compatibility="9.3.001" expanded="true" height="82" name="Set Role" origin="GENERATED_SAMPLE" width="90" x="179" y="85"> <parameter key="attribute_name" value="ChurnIndicator"/> <parameter key="target_role" value="label"/> <list key="set_additional_roles"/> </operator> </process> <?xml version="1.0" encoding="UTF-8"?><process version="9.3.001"> <operator activated="true" class="numerical_to_binominal" compatibility="9.3.001" expanded="true" height="82" name="Numerical to Binominal" origin="GENERATED_SAMPLE" width="90" x="313" y="85"> <parameter key="attribute_filter_type" value="single"/> <parameter key="attribute" value="ChurnIndicator"/> <parameter key="attributes" value=""/> <parameter key="use_except_expression" value="false"/> <parameter key="value_type" value="numeric"/> <parameter key="use_value_type_exception" value="false"/> <parameter key="except_value_type" value="real"/> <parameter key="block_type" value="value_series"/> <parameter key="use_block_type_exception" value="false"/> <parameter key="except_block_type" value="value_series_end"/> <parameter key="invert_selection" value="false"/> <parameter key="include_special_attributes" value="true"/> <parameter key="min" value="0.0"/> <parameter key="max" value="0.5"/> </operator> </process> <?xml version="1.0" encoding="UTF-8"?><process version="9.3.001"> <operator activated="true" class="concurrency:cross_validation" compatibility="8.2.000" expanded="true" height="145" name="Cross Validation" origin="GENERATED_SAMPLE" width="90" x="514" y="34"> <parameter key="split_on_batch_attribute" value="false"/> <parameter key="leave_one_out" value="false"/> <parameter key="number_of_folds" value="10"/> <parameter key="sampling_type" value="automatic"/> <parameter key="use_local_random_seed" value="true"/> <parameter key="local_random_seed" value="1992"/> <parameter key="enable_parallel_execution" value="true"/> <process expanded="true"> <operator activated="true" class="sample" compatibility="9.3.001" expanded="true" height="82" name="Sample" origin="GENERATED_SAMPLE" width="90" x="45" y="34"> <parameter key="sample" value="relative"/> <parameter key="balance_data" value="true"/> <parameter key="sample_size" value="100"/> <parameter key="sample_ratio" value="0.1"/> <parameter key="sample_probability" value="0.1"/> <list key="sample_size_per_class"/> <list key="sample_ratio_per_class"> <parameter key="true" value="1.0"/> <parameter key="false" value="0.02"/> </list> <list key="sample_probability_per_class"> <parameter key="false" value="0.02"/> <parameter key="true" value="1.0"/> </list> <parameter key="use_local_random_seed" value="false"/> <parameter key="local_random_seed" value="1992"/> </operator> <operator activated="true" class="concurrency:parallel_decision_tree" compatibility="9.3.001" expanded="true" height="82" name="Decision Tree" origin="GENERATED_SAMPLE" width="90" x="313" y="34"> <parameter key="criterion" value="gain_ratio"/> <parameter key="maximal_depth" value="20"/> <parameter key="apply_pruning" value="true"/> <parameter key="confidence" value="0.25"/> <parameter key="apply_prepruning" value="true"/> <parameter key="minimal_gain" value="0.1"/> <parameter key="minimal_leaf_size" value="2"/> <parameter key="minimal_size_for_split" value="4"/> <parameter key="number_of_prepruning_alternatives" value="3"/> </operator> <connect from_port="training set" to_op="Sample" to_port="example set input"/> <connect from_op="Sample" from_port="example set output" to_op="Decision Tree" to_port="training set"/> <connect from_op="Decision Tree" from_port="model" to_port="model"/> <portSpacing port="source_training set" spacing="0"/> <portSpacing port="sink_model" spacing="0"/> <portSpacing port="sink_through 1" spacing="0"/> <description align="left" color="yellow" colored="false" height="393" resized="false" width="217" x="10" y="10"><br> <br> <br> <br> <br> <br> <br> <br> <br> Many more customers stay than churn (hopefully!). In order for our model to learn how churners behave, we re-balance the data to focus on the case we're interested in. This is like a magnifying glass on churn!<br><br>Take a look at the 'Sample' operator.</description> <description align="left" color="green" colored="true" height="395" resized="false" width="234" x="242" y="10"><br> <br> <br> <br> <br> <br> <br> <br> <br> Let's now add a model trainer, like a Decision Tree.<br><br>Try different values for the parameters, in particular, the 'minimal gain'. The 'Wisdom of the Crowds' recommendation helps you find reasonable values.</description> </process> <process expanded="true"> <operator activated="true" class="apply_model" compatibility="9.3.001" expanded="true" height="82" name="Apply Model" origin="GENERATED_SAMPLE" width="90" x="112" y="34"> <list key="application_parameters"/> <parameter key="create_view" value="false"/> </operator> <operator activated="true" class="performance_binominal_classification" compatibility="9.3.001" expanded="true" height="82" name="Performance (Binominal Classification)" origin="GENERATED_SAMPLE" width="90" x="246" y="34"> <parameter key="manually_set_positive_class" value="false"/> <parameter key="main_criterion" value="first"/> <parameter key="accuracy" value="true"/> <parameter key="classification_error" value="false"/> <parameter key="kappa" value="false"/> <parameter key="AUC (optimistic)" value="false"/> <parameter key="AUC" value="false"/> <parameter key="AUC (pessimistic)" value="false"/> <parameter key="precision" value="false"/> <parameter key="recall" value="false"/> <parameter key="lift" value="false"/> <parameter key="fallout" value="false"/> <parameter key="f_measure" value="false"/> <parameter key="false_positive" value="false"/> <parameter key="false_negative" value="false"/> <parameter key="true_positive" value="false"/> <parameter key="true_negative" value="false"/> <parameter key="sensitivity" value="false"/> <parameter key="specificity" value="false"/> <parameter key="youden" value="false"/> <parameter key="positive_predictive_value" value="false"/> <parameter key="negative_predictive_value" value="false"/> <parameter key="psep" value="false"/> <parameter key="skip_undefined_labels" value="true"/> <parameter key="use_example_weights" value="true"/> </operator> <connect from_port="model" to_op="Apply Model" to_port="model"/> <connect from_port="test set" to_op="Apply Model" to_port="unlabelled data"/> <connect from_op="Apply Model" from_port="labelled data" to_op="Performance (Binominal Classification)" to_port="labelled data"/> <connect from_op="Performance (Binominal Classification)" from_port="performance" to_port="performance 1"/> <portSpacing port="source_model" spacing="0"/> <portSpacing port="source_test set" spacing="0"/> <portSpacing port="source_through 1" spacing="0"/> <portSpacing port="sink_test set results" spacing="0"/> <portSpacing port="sink_performance 1" spacing="0"/> <portSpacing port="sink_performance 2" spacing="0"/> <description align="left" color="red" colored="true" height="390" resized="false" width="259" x="92" y="10"><br/><br/><br/><br/><br/><br/><br/><br/><br/>The model trained on the training data is applied to the independent test data set and the model performance is calculated.<br><br>The performance values obtained on the different folds of the cross-validation are finally averaged to produce an average performance measure as well as a measure of its dispersion - which gives an estimate of the model stability when applied to different data samples.</description> </process> </operator> </process> </code><?xml version="1.0" encoding="UTF-8"?><process version="9.3.001"> <operator activated="true" class="retrieve" compatibility="9.3.001" expanded="true" height="68" name="Retrieve Customer Data" origin="GENERATED_SAMPLE" width="90" x="45" y="136"> <parameter key="repository_entry" value="//Samples/Templates/Churn Modeling/Customer Data"/> </operator> </process> <?xml version="1.0" encoding="UTF-8"?><process version="9.3.001"> <operator activated="true" class="set_role" compatibility="9.3.001" expanded="true" height="82" name="Set Role" origin="GENERATED_SAMPLE" width="90" x="179" y="85"> <parameter key="attribute_name" value="ChurnIndicator"/> <parameter key="target_role" value="label"/> <list key="set_additional_roles"/> </operator> </process> <?xml version="1.0" encoding="UTF-8"?><process version="9.3.001"> <operator activated="true" class="numerical_to_binominal" compatibility="9.3.001" expanded="true" height="82" name="Numerical to Binominal" origin="GENERATED_SAMPLE" width="90" x="313" y="85"> <parameter key="attribute_filter_type" value="single"/> <parameter key="attribute" value="ChurnIndicator"/> <parameter key="attributes" value=""/> <parameter key="use_except_expression" value="false"/> <parameter key="value_type" value="numeric"/> <parameter key="use_value_type_exception" value="false"/> <parameter key="except_value_type" value="real"/> <parameter key="block_type" value="value_series"/> <parameter key="use_block_type_exception" value="false"/> <parameter key="except_block_type" value="value_series_end"/> <parameter key="invert_selection" value="false"/> <parameter key="include_special_attributes" value="true"/> <parameter key="min" value="0.0"/> <parameter key="max" value="0.5"/> </operator> </process> <?xml version="1.0" encoding="UTF-8"?><process version="9.3.001"> <operator activated="true" class="multiply" compatibility="9.3.001" expanded="true" height="103" name="Multiply" width="90" x="380" y="187"/> </process> <?xml version="1.0" encoding="UTF-8"?><process version="9.3.001"> <operator activated="true" class="split_validation" compatibility="9.3.001" expanded="true" height="124" name="Validation" width="90" x="514" y="289"> <parameter key="create_complete_model" value="false"/> <parameter key="split" value="relative"/> <parameter key="split_ratio" value="0.7"/> <parameter key="training_set_size" value="100"/> <parameter key="test_set_size" value="-1"/> <parameter key="sampling_type" value="automatic"/> <parameter key="use_local_random_seed" value="true"/> <parameter key="local_random_seed" value="1992"/> <process expanded="true"> <operator activated="true" class="sample" compatibility="9.3.001" expanded="true" height="82" name="Sample (2)" origin="GENERATED_SAMPLE" width="90" x="45" y="34"> <parameter key="sample" value="relative"/> <parameter key="balance_data" value="true"/> <parameter key="sample_size" value="100"/> <parameter key="sample_ratio" value="0.1"/> <parameter key="sample_probability" value="0.1"/> <list key="sample_size_per_class"/> <list key="sample_ratio_per_class"> <parameter key="true" value="1.0"/> <parameter key="false" value="0.02"/> </list> <list key="sample_probability_per_class"> <parameter key="false" value="0.02"/> <parameter key="true" value="1.0"/> </list> <parameter key="use_local_random_seed" value="false"/> <parameter key="local_random_seed" value="1992"/> </operator> <operator activated="true" class="concurrency:parallel_decision_tree" compatibility="9.3.001" expanded="true" height="103" name="Decision Tree (2)" origin="GENERATED_SAMPLE" width="90" x="313" y="34"> <parameter key="criterion" value="gain_ratio"/> <parameter key="maximal_depth" value="20"/> <parameter key="apply_pruning" value="true"/> <parameter key="confidence" value="0.25"/> <parameter key="apply_prepruning" value="true"/> <parameter key="minimal_gain" value="0.1"/> <parameter key="minimal_leaf_size" value="2"/> <parameter key="minimal_size_for_split" value="4"/> <parameter key="number_of_prepruning_alternatives" value="3"/> </operator> <connect from_port="training" to_op="Sample (2)" to_port="example set input"/> <connect from_op="Sample (2)" from_port="example set output" to_op="Decision Tree (2)" to_port="training set"/> <connect from_op="Decision Tree (2)" from_port="model" to_port="model"/> <portSpacing port="source_training" spacing="0"/> <portSpacing port="sink_model" spacing="0"/> <portSpacing port="sink_through 1" spacing="0"/> </process> <process expanded="true"> <operator activated="true" class="apply_model" compatibility="9.3.001" expanded="true" height="82" name="Apply Model (2)" origin="GENERATED_SAMPLE" width="90" x="112" y="34"> <list key="application_parameters"/> <parameter key="create_view" value="false"/> </operator> <operator activated="true" class="performance_binominal_classification" compatibility="9.3.001" expanded="true" height="82" name="Performance (Binominal Classification) (2)" origin="GENERATED_SAMPLE" width="90" x="246" y="34"> <parameter key="manually_set_positive_class" value="false"/> <parameter key="main_criterion" value="first"/> <parameter key="accuracy" value="true"/> <parameter key="classification_error" value="false"/> <parameter key="kappa" value="false"/> <parameter key="AUC (optimistic)" value="false"/> <parameter key="AUC" value="false"/> <parameter key="AUC (pessimistic)" value="false"/> <parameter key="precision" value="false"/> <parameter key="recall" value="false"/> <parameter key="lift" value="false"/> <parameter key="fallout" value="false"/> <parameter key="f_measure" value="false"/> <parameter key="false_positive" value="false"/> <parameter key="false_negative" value="false"/> <parameter key="true_positive" value="false"/> <parameter key="true_negative" value="false"/> <parameter key="sensitivity" value="false"/> <parameter key="specificity" value="false"/> <parameter key="youden" value="false"/> <parameter key="positive_predictive_value" value="false"/> <parameter key="negative_predictive_value" value="false"/> <parameter key="psep" value="false"/> <parameter key="skip_undefined_labels" value="true"/> <parameter key="use_example_weights" value="true"/> </operator> <connect from_port="model" to_op="Apply Model (2)" to_port="model"/> <connect from_port="test set" to_op="Apply Model (2)" to_port="unlabelled data"/> <connect from_op="Apply Model (2)" from_port="labelled data" to_op="Performance (Binominal Classification) (2)" to_port="labelled data"/> <connect from_op="Performance (Binominal Classification) (2)" from_port="performance" to_port="averagable 1"/> <portSpacing port="source_model" spacing="0"/> <portSpacing port="source_test set" spacing="0"/> <portSpacing port="source_through 1" spacing="0"/> <portSpacing port="sink_averagable 1" spacing="0"/> <portSpacing port="sink_averagable 2" spacing="0"/> </process> </operator> </process> <?xml version="1.0" encoding="UTF-8"?><process version="9.3.001"> <operator activated="true" class="concurrency:cross_validation" compatibility="8.2.000" expanded="true" height="145" name="Cross Validation" origin="GENERATED_SAMPLE" width="90" x="514" y="34"> <parameter key="split_on_batch_attribute" value="false"/> <parameter key="leave_one_out" value="false"/> <parameter key="number_of_folds" value="10"/> <parameter key="sampling_type" value="automatic"/> <parameter key="use_local_random_seed" value="true"/> <parameter key="local_random_seed" value="1992"/> <parameter key="enable_parallel_execution" value="true"/> <process expanded="true"> <operator activated="true" class="sample" compatibility="9.3.001" expanded="true" height="82" name="Sample" origin="GENERATED_SAMPLE" width="90" x="45" y="34"> <parameter key="sample" value="relative"/> <parameter key="balance_data" value="true"/> <parameter key="sample_size" value="100"/> <parameter key="sample_ratio" value="0.1"/> <parameter key="sample_probability" value="0.1"/> <list key="sample_size_per_class"/> <list key="sample_ratio_per_class"> <parameter key="true" value="1.0"/> <parameter key="false" value="0.02"/> </list> <list key="sample_probability_per_class"> <parameter key="false" value="0.02"/> <parameter key="true" value="1.0"/> </list> <parameter key="use_local_random_seed" value="false"/> <parameter key="local_random_seed" value="1992"/> </operator> <operator activated="true" class="concurrency:parallel_decision_tree" compatibility="9.3.001" expanded="true" height="82" name="Decision Tree" origin="GENERATED_SAMPLE" width="90" x="313" y="34"> <parameter key="criterion" value="gain_ratio"/> <parameter key="maximal_depth" value="20"/> <parameter key="apply_pruning" value="true"/> <parameter key="confidence" value="0.25"/> <parameter key="apply_prepruning" value="true"/> <parameter key="minimal_gain" value="0.1"/> <parameter key="minimal_leaf_size" value="2"/> <parameter key="minimal_size_for_split" value="4"/> <parameter key="number_of_prepruning_alternatives" value="3"/> </operator> <connect from_port="training set" to_op="Sample" to_port="example set input"/> <connect from_op="Sample" from_port="example set output" to_op="Decision Tree" to_port="training set"/> <connect from_op="Decision Tree" from_port="model" to_port="model"/> <portSpacing port="source_training set" spacing="0"/> <portSpacing port="sink_model" spacing="0"/> <portSpacing port="sink_through 1" spacing="0"/> <description align="left" color="yellow" colored="false" height="393" resized="false" width="217" x="10" y="10"><br> <br> <br> <br> <br> <br> <br> <br> <br> Many more customers stay than churn (hopefully!). In order for our model to learn how churners behave, we re-balance the data to focus on the case we're interested in. This is like a magnifying glass on churn!<br><br>Take a look at the 'Sample' operator.</description> <description align="left" color="green" colored="true" height="395" resized="false" width="234" x="242" y="10"><br> <br> <br> <br> <br> <br> <br> <br> <br> Let's now add a model trainer, like a Decision Tree.<br><br>Try different values for the parameters, in particular, the 'minimal gain'. The 'Wisdom of the Crowds' recommendation helps you find reasonable values.</description> </process> <process expanded="true"> <operator activated="true" class="apply_model" compatibility="9.3.001" expanded="true" height="82" name="Apply Model" origin="GENERATED_SAMPLE" width="90" x="112" y="34"> <list key="application_parameters"/> <parameter key="create_view" value="false"/> </operator> <operator activated="true" class="performance_binominal_classification" compatibility="9.3.001" expanded="true" height="82" name="Performance (Binominal Classification)" origin="GENERATED_SAMPLE" width="90" x="246" y="34"> <parameter key="manually_set_positive_class" value="false"/> <parameter key="main_criterion" value="first"/> <parameter key="accuracy" value="true"/> <parameter key="classification_error" value="false"/> <parameter key="kappa" value="false"/> <parameter key="AUC (optimistic)" value="false"/> <parameter key="AUC" value="false"/> <parameter key="AUC (pessimistic)" value="false"/> <parameter key="precision" value="false"/> <parameter key="recall" value="false"/> <parameter key="lift" value="false"/> <parameter key="fallout" value="false"/> <parameter key="f_measure" value="false"/> <parameter key="false_positive" value="false"/> <parameter key="false_negative" value="false"/> <parameter key="true_positive" value="false"/> <parameter key="true_negative" value="false"/> <parameter key="sensitivity" value="false"/> <parameter key="specificity" value="false"/> <parameter key="youden" value="false"/> <parameter key="positive_predictive_value" value="false"/> <parameter key="negative_predictive_value" value="false"/> <parameter key="psep" value="false"/> <parameter key="skip_undefined_labels" value="true"/> <parameter key="use_example_weights" value="true"/> </operator> <connect from_port="model" to_op="Apply Model" to_port="model"/> <connect from_port="test set" to_op="Apply Model" to_port="unlabelled data"/> <connect from_op="Apply Model" from_port="labelled data" to_op="Performance (Binominal Classification)" to_port="labelled data"/> <connect from_op="Performance (Binominal Classification)" from_port="performance" to_port="performance 1"/> <portSpacing port="source_model" spacing="0"/> <portSpacing port="source_test set" spacing="0"/> <portSpacing port="source_through 1" spacing="0"/> <portSpacing port="sink_test set results" spacing="0"/> <portSpacing port="sink_performance 1" spacing="0"/> <portSpacing port="sink_performance 2" spacing="0"/> <description align="left" color="red" colored="true" height="390" resized="false" width="259" x="92" y="10"><br/><br/><br/><br/><br/><br/><br/><br/><br/>The model trained on the training data is applied to the independent test data set and the model performance is calculated.<br><br>The performance values obtained on the different folds of the cross-validation are finally averaged to produce an average performance measure as well as a measure of its dispersion - which gives an estimate of the model stability when applied to different data samples.</description> </process> </operator> </process> </pre><div>****This script below is for only cross validation</div><pre class="CodeBlock"><code>
Tagged:
0
Best Answer
-
varunm1 Member Posts: 1,207
Unicorn
Hello @Atiah
In cross-validation and split validation the data is being divided into different train and test sets, if you don't set the seed the subsets might change based on the random index numbers generated by your computer during different executions. To get the same train and test sets every time you open the software and execute, you need to set seed. Basically, even if a single sample changes in your train and test set, it might impact your performance.
If you need more info please inform here.Regards,
Varun
https://www.varunmandalapu.com/Be Safe. Follow precautions and Maintain Social Distancing
5
Answers
Did you selected set local random seed in cross validation and split validation operators? If you do this, your results doesn't change.
Varun
https://www.varunmandalapu.com/
Be Safe. Follow precautions and Maintain Social Distancing
Thanks
Varun
https://www.varunmandalapu.com/
Be Safe. Follow precautions and Maintain Social Distancing
After checking the XMLs, the XMLs seems to be invalid because there are multiple lines with :
:
@Atiah Can you export your processes in .rmp files (via File -> Export Process)
Thanks,
Regards,
Lionel
https://community.rapidminer.com/discussion/56127/testing-of-automodel#latest
Varun
https://www.varunmandalapu.com/
Be Safe. Follow precautions and Maintain Social Distancing
For now I recommend you attach the .rmp file to a discussion rather than cut-and-paste. It will import much better.
Scott
it work like that, and what is local random seed exactly?
Thank you