The Altair Community is migrating to a new platform to provide a better experience for you. In preparation for the migration, the Altair Community is on read-only mode from October 28 - November 6, 2024. Technical support via cases will continue to work as is. For any urgent requests from Students/Faculty members, please submit the form linked here
How to train fast the Hybrid or combined models ?
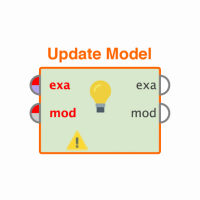

I am Training a hybrid or combined model of KNN and Decision Tree with 10 Fold cross-validation. Unable to train it because of training time! any alternate way to train a model with less time !!!! The XML and picture of the process are attached below
<?xml version="1.0" encoding="UTF-8"?><process version="9.5.001">
<?xml version="1.0" encoding="UTF-8"?><process version="9.5.001">
<context>
<input/>
<output/>
<macros/>
</context>
<operator activated="true" class="process" compatibility="6.0.002" expanded="true" name="Process" origin="GENERATED_TUTORIAL">
<parameter key="logverbosity" value="init"/>
<parameter key="random_seed" value="2001"/>
<parameter key="send_mail" value="never"/>
<parameter key="notification_email" value=""/>
<parameter key="process_duration_for_mail" value="30"/>
<parameter key="encoding" value="SYSTEM"/>
<process expanded="true">
<operator activated="true" class="retrieve" compatibility="9.5.001" expanded="true" height="68" name="Retrieve Violation" width="90" x="45" y="136">
<parameter key="repository_entry" value="//Temporary Repository/Violation"/>
</operator>
<operator activated="true" class="set_role" compatibility="9.5.001" expanded="true" height="82" name="Set Role" width="90" x="179" y="136">
<parameter key="attribute_name" value="Violation"/>
<parameter key="target_role" value="label"/>
<list key="set_additional_roles"/>
</operator>
<operator activated="true" class="select_attributes" compatibility="9.5.001" expanded="true" height="82" name="Select Attributes" width="90" x="313" y="136">
<parameter key="attribute_filter_type" value="subset"/>
<parameter key="attribute" value=""/>
<parameter key="attributes" value="address|Hour |latitude|longitude|Month|PH /Off PH|road type|Season|T.V.B code |type of vehicle number|vehicle number|vehicle Type|vehicle use|Violation|WD/WRD|Weekday|time"/>
<parameter key="use_except_expression" value="false"/>
<parameter key="value_type" value="attribute_value"/>
<parameter key="use_value_type_exception" value="false"/>
<parameter key="except_value_type" value="time"/>
<parameter key="block_type" value="attribute_block"/>
<parameter key="use_block_type_exception" value="false"/>
<parameter key="except_block_type" value="value_matrix_row_start"/>
<parameter key="invert_selection" value="false"/>
<parameter key="include_special_attributes" value="false"/>
</operator>
<operator activated="true" class="concurrency:cross_validation" compatibility="9.5.001" expanded="true" height="145" name="Cross Validation" width="90" x="447" y="136">
<parameter key="split_on_batch_attribute" value="false"/>
<parameter key="leave_one_out" value="false"/>
<parameter key="number_of_folds" value="10"/>
<parameter key="sampling_type" value="stratified sampling"/>
<parameter key="use_local_random_seed" value="false"/>
<parameter key="local_random_seed" value="1992"/>
<parameter key="enable_parallel_execution" value="true"/>
<process expanded="true">
<operator activated="true" class="multiply" compatibility="9.5.001" expanded="true" height="103" name="Multiply" width="90" x="11" y="34"/>
<operator activated="true" class="concurrency:parallel_decision_tree" compatibility="9.5.001" expanded="true" height="103" name="Decision Tree" width="90" x="179" y="34">
<parameter key="criterion" value="gain_ratio"/>
<parameter key="maximal_depth" value="10"/>
<parameter key="apply_pruning" value="true"/>
<parameter key="confidence" value="0.1"/>
<parameter key="apply_prepruning" value="true"/>
<parameter key="minimal_gain" value="0.01"/>
<parameter key="minimal_leaf_size" value="2"/>
<parameter key="minimal_size_for_split" value="4"/>
<parameter key="number_of_prepruning_alternatives" value="3"/>
</operator>
<operator activated="true" class="k_nn" compatibility="9.5.001" expanded="true" height="82" name="k-NN" width="90" x="179" y="238">
<parameter key="k" value="5"/>
<parameter key="weighted_vote" value="true"/>
<parameter key="measure_types" value="MixedMeasures"/>
<parameter key="mixed_measure" value="MixedEuclideanDistance"/>
<parameter key="nominal_measure" value="NominalDistance"/>
<parameter key="numerical_measure" value="EuclideanDistance"/>
<parameter key="divergence" value="GeneralizedIDivergence"/>
<parameter key="kernel_type" value="radial"/>
<parameter key="kernel_gamma" value="1.0"/>
<parameter key="kernel_sigma1" value="1.0"/>
<parameter key="kernel_sigma2" value="0.0"/>
<parameter key="kernel_sigma3" value="2.0"/>
<parameter key="kernel_degree" value="3.0"/>
<parameter key="kernel_shift" value="1.0"/>
<parameter key="kernel_a" value="1.0"/>
<parameter key="kernel_b" value="0.0"/>
</operator>
<operator activated="true" class="radoop:combine_models" compatibility="9.3.000" expanded="true" height="103" name="Combine Models" width="90" x="313" y="136"/>
<connect from_port="training set" to_op="Multiply" to_port="input"/>
<connect from_op="Multiply" from_port="output 1" to_op="Decision Tree" to_port="training set"/>
<connect from_op="Multiply" from_port="output 2" to_op="k-NN" to_port="training set"/>
<connect from_op="Decision Tree" from_port="model" to_op="Combine Models" to_port="model input 1"/>
<connect from_op="k-NN" from_port="model" to_op="Combine Models" to_port="model input 2"/>
<connect from_op="Combine Models" from_port="model output" to_port="model"/>
<portSpacing port="source_training set" spacing="0"/>
<portSpacing port="sink_model" spacing="0"/>
<portSpacing port="sink_through 1" spacing="0"/>
</process>
<process expanded="true">
<operator activated="true" class="apply_model" compatibility="9.5.001" expanded="true" height="82" name="Apply Model" width="90" x="45" y="34">
<list key="application_parameters"/>
<parameter key="create_view" value="false"/>
</operator>
<operator activated="true" class="performance_classification" compatibility="9.5.001" expanded="true" height="82" name="Performance" width="90" x="179" y="34">
<parameter key="main_criterion" value="first"/>
<parameter key="accuracy" value="true"/>
<parameter key="classification_error" value="false"/>
<parameter key="kappa" value="false"/>
<parameter key="weighted_mean_recall" value="false"/>
<parameter key="weighted_mean_precision" value="false"/>
<parameter key="spearman_rho" value="false"/>
<parameter key="kendall_tau" value="false"/>
<parameter key="absolute_error" value="false"/>
<parameter key="relative_error" value="false"/>
<parameter key="relative_error_lenient" value="false"/>
<parameter key="relative_error_strict" value="false"/>
<parameter key="normalized_absolute_error" value="false"/>
<parameter key="root_mean_squared_error" value="false"/>
<parameter key="root_relative_squared_error" value="false"/>
<parameter key="squared_error" value="false"/>
<parameter key="correlation" value="false"/>
<parameter key="squared_correlation" value="false"/>
<parameter key="cross-entropy" value="false"/>
<parameter key="margin" value="false"/>
<parameter key="soft_margin_loss" value="false"/>
<parameter key="logistic_loss" value="false"/>
<parameter key="skip_undefined_labels" value="true"/>
<parameter key="use_example_weights" value="true"/>
<list key="class_weights"/>
</operator>
<connect from_port="model" to_op="Apply Model" to_port="model"/>
<connect from_port="test set" to_op="Apply Model" to_port="unlabelled data"/>
<connect from_op="Apply Model" from_port="labelled data" to_op="Performance" to_port="labelled data"/>
<connect from_op="Performance" from_port="performance" to_port="performance 1"/>
<portSpacing port="source_model" spacing="0"/>
<portSpacing port="source_test set" spacing="0"/>
<portSpacing port="source_through 1" spacing="0"/>
<portSpacing port="sink_test set results" spacing="0"/>
<portSpacing port="sink_performance 1" spacing="0"/>
<portSpacing port="sink_performance 2" spacing="0"/>
</process>
</operator>
<connect from_op="Retrieve Violation" from_port="output" to_op="Set Role" to_port="example set input"/>
<connect from_op="Set Role" from_port="example set output" to_op="Select Attributes" to_port="example set input"/>
<connect from_op="Select Attributes" from_port="example set output" to_op="Cross Validation" to_port="example set"/>
<connect from_op="Cross Validation" from_port="performance 1" to_port="result 1"/>
<portSpacing port="source_input 1" spacing="0"/>
<portSpacing port="sink_result 1" spacing="0"/>
<portSpacing port="sink_result 2" spacing="90"/>
</process>
</operator>
</process>
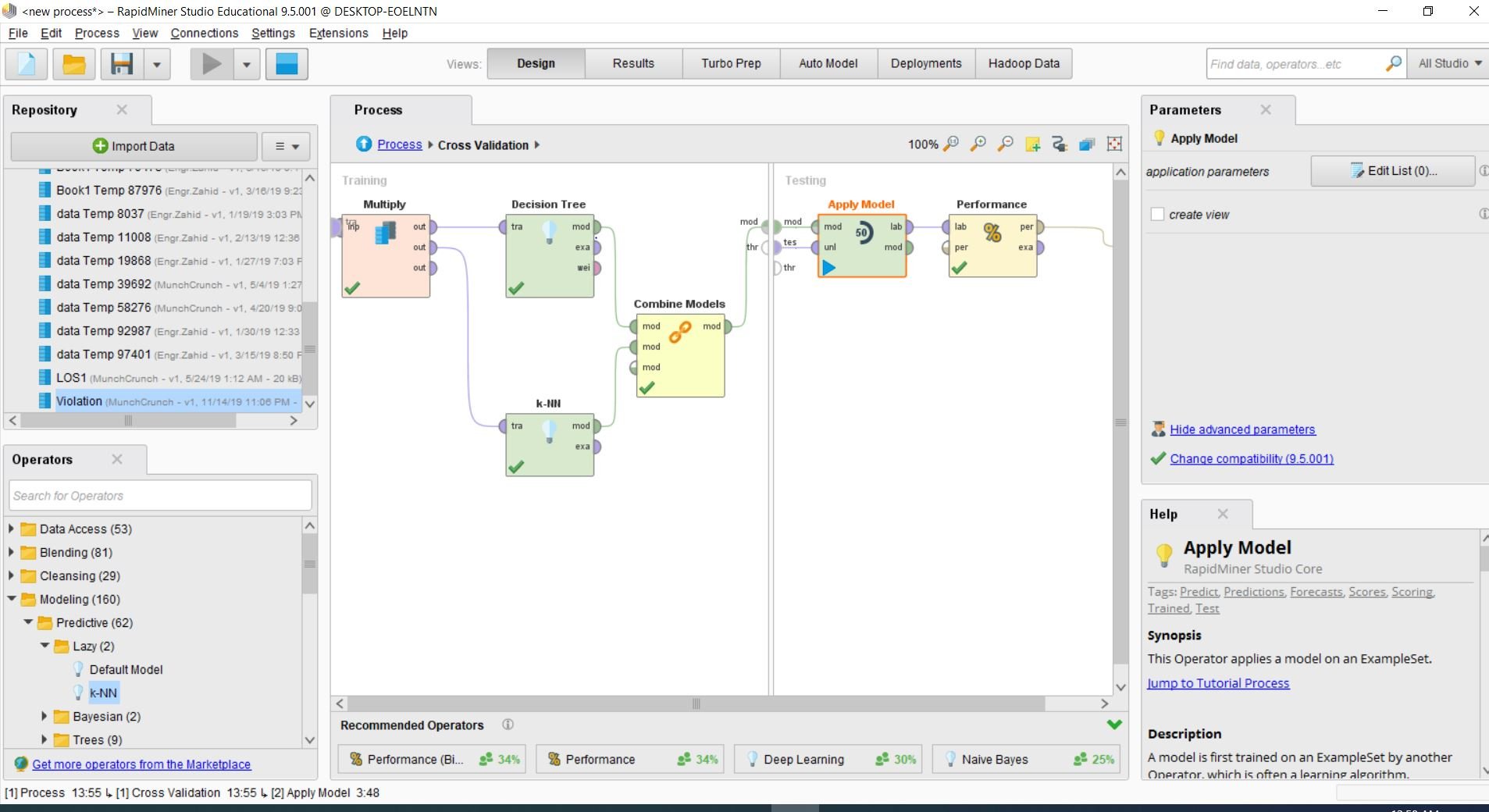
0
Answers
Processing time mainly depends on the number of logical cores available with your license and in your processor. If you have an unlimited access license, then a processor with a high number of logical cores will help speed up processes. This is due to the capability of parallel execution in the case of a cross-validation operator in your process.
Reducing the number of folds in cross-validation also might improve processing times as the number of models that need to be built will be decreased.
Varun
https://www.varunmandalapu.com/
Be Safe. Follow precautions and Maintain Social Distancing