The Altair Community is migrating to a new platform to provide a better experience for you. In preparation for the migration, the Altair Community is on read-only mode from October 28 - November 6, 2024. Technical support via cases will continue to work as is. For any urgent requests from Students/Faculty members, please submit the form linked here
Deep Learning suggestions/guidance
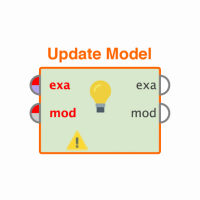

in Help
Hello,
So I have an assignment where I'm constructing a deep learning model to achieve, hopefully, a classification accuracy of at least 63%. I've started with a few fully-connected layers, then some CNN/RNN combinations and to no avail am able to achieve a rate of about 25%. Can someone provide any suggestions or feedback on the model? Also, for this assignment I am unable to use the auto-model(which I have already and it achieved a rate of 63% but am unable to replicate).
XML below(and data attached):
So I have an assignment where I'm constructing a deep learning model to achieve, hopefully, a classification accuracy of at least 63%. I've started with a few fully-connected layers, then some CNN/RNN combinations and to no avail am able to achieve a rate of about 25%. Can someone provide any suggestions or feedback on the model? Also, for this assignment I am unable to use the auto-model(which I have already and it achieved a rate of 63% but am unable to replicate).
XML below(and data attached):
<?xml version="1.0" encoding="UTF-8"?><process version="9.6.000">
<context>
<input/>
<output/>
<macros/>
</context>
<operator activated="true" class="process" compatibility="9.6.000" expanded="true" name="Process">
<parameter key="logverbosity" value="init"/>
<parameter key="random_seed" value="2001"/>
<parameter key="send_mail" value="never"/>
<parameter key="notification_email" value=""/>
<parameter key="process_duration_for_mail" value="30"/>
<parameter key="encoding" value="SYSTEM"/>
<process expanded="true">
<operator activated="true" class="retrieve" compatibility="9.6.000" expanded="true" height="68" name="Retrieve Tweets_sequence" width="90" x="45" y="34">
<parameter key="repository_entry" value="//Local Repository/data/Tweets_sequence"/>
</operator>
<operator activated="true" class="subprocess" compatibility="9.6.000" expanded="true" height="103" name="Subprocess" width="90" x="179" y="34">
<process expanded="true">
<operator activated="true" class="select_attributes" compatibility="9.6.000" expanded="true" height="82" name="Select Attributes" width="90" x="45" y="34">
<parameter key="attribute_filter_type" value="all"/>
<parameter key="attribute" value="sentiment"/>
<parameter key="attributes" value=""/>
<parameter key="use_except_expression" value="false"/>
<parameter key="value_type" value="numeric"/>
<parameter key="use_value_type_exception" value="false"/>
<parameter key="except_value_type" value="time"/>
<parameter key="block_type" value="attribute_block"/>
<parameter key="use_block_type_exception" value="false"/>
<parameter key="except_block_type" value="value_matrix_row_start"/>
<parameter key="invert_selection" value="false"/>
<parameter key="include_special_attributes" value="true"/>
</operator>
<operator activated="true" class="set_role" compatibility="9.6.000" expanded="true" height="82" name="Set Role" width="90" x="246" y="85">
<parameter key="attribute_name" value="sentiment"/>
<parameter key="target_role" value="label"/>
<list key="set_additional_roles"/>
</operator>
<operator activated="true" class="split_data" compatibility="9.6.000" expanded="true" height="103" name="Split Data" width="90" x="447" y="85">
<enumeration key="partitions">
<parameter key="ratio" value="0.8"/>
<parameter key="ratio" value="0.2"/>
</enumeration>
<parameter key="sampling_type" value="automatic"/>
<parameter key="use_local_random_seed" value="false"/>
<parameter key="local_random_seed" value="1992"/>
</operator>
<connect from_port="in 1" to_op="Select Attributes" to_port="example set input"/>
<connect from_op="Select Attributes" from_port="example set output" to_op="Set Role" to_port="example set input"/>
<connect from_op="Set Role" from_port="example set output" to_op="Split Data" to_port="example set"/>
<connect from_op="Split Data" from_port="partition 1" to_port="out 1"/>
<connect from_op="Split Data" from_port="partition 2" to_port="out 2"/>
<portSpacing port="source_in 1" spacing="0"/>
<portSpacing port="source_in 2" spacing="0"/>
<portSpacing port="sink_out 1" spacing="0"/>
<portSpacing port="sink_out 2" spacing="0"/>
<portSpacing port="sink_out 3" spacing="0"/>
</process>
</operator>
<operator activated="true" class="deeplearning:dl4j_sequential_neural_network" compatibility="0.9.003" expanded="true" height="103" name="Deep Learning" width="90" x="313" y="340">
<parameter key="loss_function" value="Mean Absolute Error (Regression)"/>
<parameter key="epochs" value="10"/>
<parameter key="use_miniBatch" value="true"/>
<parameter key="batch_size" value="32"/>
<parameter key="updater" value="Adam"/>
<parameter key="learning_rate" value="0.01"/>
<parameter key="momentum" value="0.9"/>
<parameter key="rho" value="0.95"/>
<parameter key="epsilon" value="1.0E-6"/>
<parameter key="beta1" value="0.9"/>
<parameter key="beta2" value="0.999"/>
<parameter key="RMSdecay" value="0.95"/>
<parameter key="weight_initialization" value="ReLU"/>
<parameter key="bias_initialization" value="0.0"/>
<parameter key="use_regularization" value="false"/>
<parameter key="l1_strength" value="0.1"/>
<parameter key="l2_strength" value="0.1"/>
<parameter key="optimization_method" value="Stochastic Gradient Descent"/>
<parameter key="backpropagation" value="Standard"/>
<parameter key="backpropagation_length" value="50"/>
<parameter key="infer_input_shape" value="true"/>
<parameter key="network_type" value="Simple Neural Network"/>
<parameter key="log_each_epoch" value="true"/>
<parameter key="epochs_per_log" value="10"/>
<parameter key="use_local_random_seed" value="false"/>
<parameter key="local_random_seed" value="1992"/>
<process expanded="true">
<operator activated="true" class="deeplearning:dl4j_dense_layer" compatibility="0.9.003" expanded="true" height="68" name="Add Fully-Connected Layer (3)" width="90" x="112" y="85">
<parameter key="number_of_neurons" value="9"/>
<parameter key="activation_function" value="ReLU (Rectified Linear Unit)"/>
<parameter key="use_dropout" value="false"/>
<parameter key="dropout_rate" value="0.25"/>
<parameter key="overwrite_networks_weight_initialization" value="false"/>
<parameter key="weight_initialization" value="Normal"/>
<parameter key="overwrite_networks_bias_initialization" value="false"/>
<parameter key="bias_initialization" value="0.0"/>
</operator>
<operator activated="true" class="deeplearning:dl4j_convolutional_layer" compatibility="0.9.003" expanded="true" height="68" name="Add Convolutional Layer" width="90" x="313" y="85">
<parameter key="number_of_activation_maps" value="128"/>
<parameter key="kernel_size" value="2.2"/>
<parameter key="stride_size" value="2.2"/>
<parameter key="activation_function" value="ReLU (Rectified Linear Unit)"/>
<parameter key="use_dropout" value="false"/>
<parameter key="dropout_rate" value="0.25"/>
<parameter key="overwrite_networks_weight_initialization" value="false"/>
<parameter key="weight_initialization" value="Normal"/>
<parameter key="overwrite_networks_bias_initialization" value="false"/>
<parameter key="bias_initialization" value="0.0"/>
</operator>
<operator activated="true" class="deeplearning:dl4j_lstm_layer" compatibility="0.9.003" expanded="true" height="68" name="Add LSTM Layer" width="90" x="447" y="85">
<parameter key="neurons" value="32"/>
<parameter key="gate_activation" value="ReLU (Rectified Linear Unit)"/>
<parameter key="forget_gate_bias_initialization" value="1.0"/>
</operator>
<operator activated="true" class="deeplearning:dl4j_pooling_layer" compatibility="0.9.003" expanded="true" height="68" name="Add Pooling Layer" width="90" x="648" y="85">
<parameter key="Pooling Method" value="max"/>
<parameter key="PNorm Value" value="1.0"/>
<parameter key="Kernel Size" value="2.2"/>
<parameter key="Stride Size" value="1.1"/>
</operator>
<operator activated="true" class="deeplearning:dl4j_dense_layer" compatibility="0.9.003" expanded="true" height="68" name="Add Fully-Connected Layer" width="90" x="782" y="187">
<parameter key="number_of_neurons" value="9"/>
<parameter key="activation_function" value="Softmax"/>
<parameter key="use_dropout" value="false"/>
<parameter key="dropout_rate" value="0.25"/>
<parameter key="overwrite_networks_weight_initialization" value="false"/>
<parameter key="weight_initialization" value="Normal"/>
<parameter key="overwrite_networks_bias_initialization" value="false"/>
<parameter key="bias_initialization" value="0.0"/>
</operator>
<connect from_port="layerArchitecture" to_op="Add Fully-Connected Layer (3)" to_port="layerArchitecture"/>
<connect from_op="Add Fully-Connected Layer (3)" from_port="layerArchitecture" to_op="Add Convolutional Layer" to_port="layerArchitecture"/>
<connect from_op="Add Convolutional Layer" from_port="layerArchitecture" to_op="Add LSTM Layer" to_port="layerArchitecture"/>
<connect from_op="Add LSTM Layer" from_port="layerArchitecture" to_op="Add Pooling Layer" to_port="layerArchitecture"/>
<connect from_op="Add Pooling Layer" from_port="layerArchitecture" to_op="Add Fully-Connected Layer" to_port="layerArchitecture"/>
<connect from_op="Add Fully-Connected Layer" from_port="layerArchitecture" to_port="layerArchitecture"/>
<portSpacing port="source_layerArchitecture" spacing="0"/>
<portSpacing port="sink_layerArchitecture" spacing="0"/>
</process>
</operator>
<operator activated="true" class="apply_model" compatibility="9.6.000" expanded="true" height="82" name="Apply Model" width="90" x="514" y="187">
<list key="application_parameters"/>
<parameter key="create_view" value="false"/>
</operator>
<operator activated="true" class="performance_regression" compatibility="9.6.000" expanded="true" height="82" name="Performance (2)" width="90" x="782" y="187">
<parameter key="main_criterion" value="first"/>
<parameter key="root_mean_squared_error" value="true"/>
<parameter key="absolute_error" value="true"/>
<parameter key="relative_error" value="true"/>
<parameter key="relative_error_lenient" value="false"/>
<parameter key="relative_error_strict" value="false"/>
<parameter key="normalized_absolute_error" value="false"/>
<parameter key="root_relative_squared_error" value="false"/>
<parameter key="squared_error" value="false"/>
<parameter key="correlation" value="false"/>
<parameter key="squared_correlation" value="false"/>
<parameter key="prediction_average" value="false"/>
<parameter key="spearman_rho" value="false"/>
<parameter key="kendall_tau" value="false"/>
<parameter key="skip_undefined_labels" value="true"/>
<parameter key="use_example_weights" value="true"/>
</operator>
<connect from_op="Retrieve Tweets_sequence" from_port="output" to_op="Subprocess" to_port="in 1"/>
<connect from_op="Subprocess" from_port="out 1" to_op="Deep Learning" to_port="training set"/>
<connect from_op="Subprocess" from_port="out 2" to_op="Apply Model" to_port="unlabelled data"/>
<connect from_op="Deep Learning" from_port="model" to_op="Apply Model" to_port="model"/>
<connect from_op="Apply Model" from_port="labelled data" to_op="Performance (2)" to_port="labelled data"/>
<connect from_op="Performance (2)" from_port="performance" to_port="result 1"/>
<connect from_op="Performance (2)" from_port="example set" to_port="result 2"/>
<portSpacing port="source_input 1" spacing="0"/>
<portSpacing port="sink_result 1" spacing="0"/>
<portSpacing port="sink_result 2" spacing="0"/>
<portSpacing port="sink_result 3" spacing="0"/>
</process>
</operator>
</process>
Tagged:
0
Best Answer
-
hughesfleming68 Member Posts: 323
Unicorn
Is there a reason why you setup your deep net this way? I would start with a couple of Fully connected layers and an output layer and then add complexity from there. Experiment with CNN and LSTM separately. You will probably get better results with a simple network by adjusting your learning rate and epochs. Experiment with different activation functions and don't assume that ReLU is the right one for all problems.
There are hundreds of examples of deep net architectures on the net. Beginners should follow a template until you have the time and understanding to create your own. It tends to be a time consuming evolutionary process so you have to be ready for that.6