The Altair Community is migrating to a new platform to provide a better experience for you. In preparation for the migration, the Altair Community is on read-only mode from October 28 - November 6, 2024. Technical support via cases will continue to work as is. For any urgent requests from Students/Faculty members, please submit the form linked here
Error when using Convolutional Layer: Message: New shape length doesn't match original length
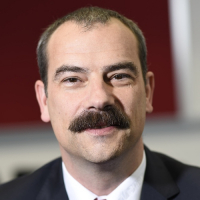

in Help
Hi,
I am trying to setup a simple Deep Learning process using the Deep Learning extension and the MNIST dataset as CSVs of grey values from Kaggle. If I just use two fully connected layers inside the Deep Learning operator everything works, but as soon as I add a convolutional layer and a pooling layer, the apply model steps fails with an error message:
Exception: org.nd4j.linalg.exception.ND4JIllegalStateException
Message: New shape length doesn't match original length: [0] vs [6584816]. Original shape: [8399, 784] New Shape: [33601, 0, 784]
The test dataset is the result of a split operator which is used to have 80% (33601 records) of the data as training data and 20% (8399 records) as test data.
What am I doing wrong? Any help is highly appreciated
Friedemann
Tagged:
0
Best Answer
-
Mate Employee-RapidMiner, Member Posts: 14
RM Team Member
Well, I have never used a dataset where 3-channel (e.g.: RGB) images were put into a single array, but I did conduct a little test now:
this is one of the MNIST images reduced to 4x4 and converted to 3-channel image (I stopped the process and looked directly at the data, how those MNIST png images look like under the hood, so this is now not the ExampleSet use-case, but rather the tutorial process where we deal with actual images files):
that means, the tensor is 4D because we are always talking about a collection of samples (in this case a single image), and the 3D tensor inside will contain 2D matrices for each channel/depth.
That means, this is the desired format which I'd like to get to, if I do convert my manual data set to a 3-channel one, as I did for 1-channel in my previous message.3 channels:[[0, 1.0000, 0, 0, 1.0000, 0, 0, 1.0000, 0, 0, 2.0000, 0, 0, 2.0000, 0, 0, 2.0000, 0, 0, 3.0000, 0, 0, 3.0000, 0, 0, 3.0000, 0]]
becomes[[[[ 0, 1.0000, 0],[ 0, 1.0000, 0],[ 0, 1.0000, 0]],[[ 0, 2.0000, 0],[ 0, 2.0000, 0],[ 0, 2.0000, 0]],[[ 0, 3.0000, 0],[ 0, 3.0000, 0],[ 0, 3.0000, 0]]]]
So, this is a 3x3x3 image (height: 3, width: 3, depth/channel: 3).
As you can see, if you put your data in a row, in the right order (first channel, second channel, third channel) and do set the correct Input Shape parameter for the network operator, you can even deal with multi-channel images sitting in a single ExampleSet row.0
Answers
as a first suggestion, I'd say, have a look at one of our tutorial processes, since it also deals with the MNIST use-case.
"Add Convolutional Layer --> Tutorial Process --> MNIST classification"
(of course we do not provide the data, but you already have it so, that's no problem for you)
The error btw occurs due to the fact that a fully-connected layer is fed into a convolutional one.
My intuition tells me, that this shouldn't even be automatically possible since CNN-s work on multi-dimensional data, right ?
So, the very least we should do for making a potential automatic transformation possible, is to configure how to shape the 1-dimensional output of given fully-connected layer thus making it digestible for an upcoming convolutional layer, shouldn't we ?
At least I think so.
+ I am pretty sure, we don't provide that functionality and I guess it would also be a bit strange and unusual, at least based on what I've seen in other CNN models (with the help of the "Import Existing Model" you can check out the architectures of numerous famous models).
Kind regards,
Mate
- Yes, the tutorial process uses a different "format" (as you also concluded that in your follow-up response, so this part of my original answer becomes irrelevant since you are using CSV format or at least you were using that).
- You could have sticked with your original data-format (1D, 28x28 columns/attributes/features for a single image), but would have needed to correctly devise your network then.
- I'll be honest with you, I only trained and tested on a reduced set.
Kind regards,I'm not sure if the training worked, though. Can you see training scores in your log window while doing the training ? Sometimes instead of an immediate error, you'll only get warnings informing you that the network could not be trained in the given epoch.
My answer was rather implying that your network was not going to work, regardless of the "format" of your data:
putting a Dense Layer in front of a Convolutional Layer is problematic. That was what I intended to point out.
Took 100 images from each class thus creating a 1000-image large, distinct dataset for training and testing respectively.
Since I also used CPU for training I did not want to deal with 60.000 images.
When I did this, the tutorial process achieved ~89% accuracy, training only taking 1 minute 20 secs (I'm on a laptop).
Even if I then apply the model to the entire test dataset (around 10.000 images), it achieves around 90% accuracy. I'd say the model learnt pretty successfully how to distinguish those numbers (of course it can still be made more robust and better, I think LeNet achieves around 97%, if I remember it correctly).
Mate
CNN using 1D input (784 + 1 columns).
Mind the Input Shape setting on the Deep Learning model operator.
- One question was how to use CNNs with the csv version of the MNIST data.
- The actual question in this thread is how to prepare a tensor from an example set containing data like for sequential patterns for usage with a "regular" 2-D CNNs (label, image, pixel_values_of_line)?
CheersThat has been answered by your response. In a previous response I was told that I do need two-dimensional data for a CNN but it seems that if you use the "manual input shape specification" the 1D version of the DL4J package is invoked.
Update:
I read the explanation of "Convolutional Flattened" multiple times now. I guess my understanding was incorrect. The explanation says that the four dimensional input is converted to two dimensions. What kind of four dimensional input is expected? The CSV input is 2-dimensional (label, pixel_values). Do I need data like in the next question (label, image, pixel_values_of_line) and the 2D kernel will be invoked?
I guess that I need to select the "Convolutional" shape option but which format of the example set is expected then?
However, when it comes to multidimensional signals, like images and videos, 2D and 3D convolutions take over.
I think this is what I was referring to when I said, you'd need multi-dimensional data, if you were to use convolutional layers for image processing.
Now, in my above process, the 2D convolutional layer is being used and that was what I was previously also looking for, but got confused by the documentation. Hence "configure how to shape the 1-dimensional output of given fully-connected layer thus making it digestible for an upcoming convolutional layer". Except that now I removed the fully-connected/dense layer and just needed to make the 1D data directly digestible for the convolutional one.
I basically told DL4j, that "hey, this a 784 elements in an array, but I want to have it as 28x28. I literally just reshaped my data before consuming it in the convolutional layer. Under the hood this works by using pre-processors, in this case: FeedForwardToCnnPreProcessor.
I think for multivariate time-series involving convolutional layers we don't necessarily have a tutorial process, but that is certainly possible, especially with the right selection of input shape. Although, in this case, I'd definitely use one of the recurrent options.
Kind regards,
Mate
Friedemann
I am not sure if I understand the rest of your question, but here is an example what is happening in the background:
Original data, a collection of rows (~array of arrays, this corresponds to an ExampleSet with a single row):
Now said pre-processor takes the above data and reshapes it into this:
Let's say the above "image" stands for the number 1.
Number of channels / depth was set to 1 (in my sample process as well), since our data is grayscale.
Now this format can directly be processed by the convolutional layer.