The Altair Community is migrating to a new platform to provide a better experience for you. In preparation for the migration, the Altair Community is on read-only mode from October 28 - November 6, 2024. Technical support via cases will continue to work as is. For any urgent requests from Students/Faculty members, please submit the form linked here
The Decision Tree gave impossible result
I just trained a machine using a Decision Tree, that reached an F-score of 99,7%.
Which sounds good until you hear, that naive bayes only got 66,4%
the highest score on that dataset I found was 98,2% using deep learning
The highest CREDIBLE score I found on that dataset was 78,5%
The design is based off of this video:
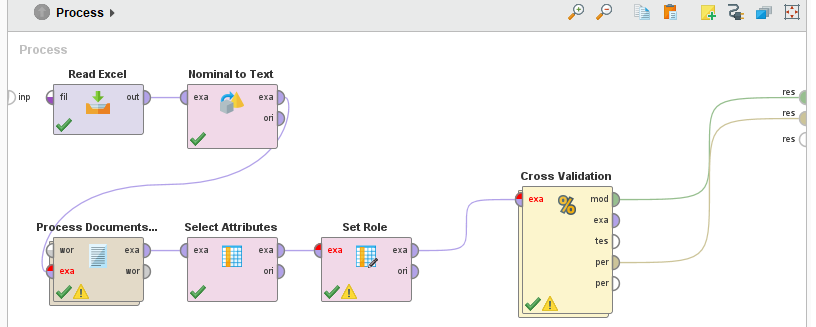
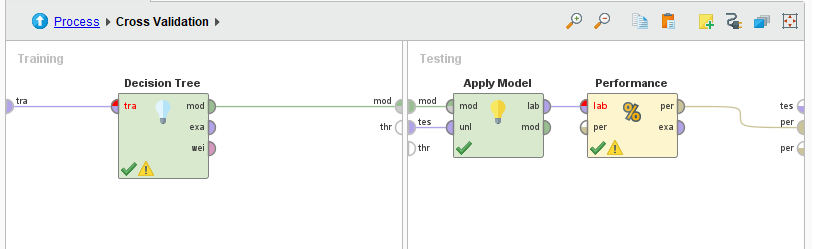
All I did was replace the Naive Bayes operator in the Crossvalidation with the Decision Tree operator.
Even with 10-fold Crossvalidation I should still not get much more than 70%...
The immediate cause of the high score is, that for some reason there is a strong correlations between the label and the id, however I do not know how to limit which collumns the algorithm uses.
The question is, what did I do wrong? How do I make it right?
0
Best Answer
-
MartinLiebig Administrator, Moderator, Employee-RapidMiner, RapidMiner Certified Analyst, RapidMiner Certified Expert, University Professor Posts: 3,533
RM Data Scientist
often its just because the two sets for the two classes got append. So the first half of the data set is true, the second half is false?Otherwise: Often ids correlate with dates, which correlate with the label.What you want to do is either use Select Attributes and remove the id or set role and set the role of id to id.Best,Martin- Sr. Director Data Solutions, Altair RapidMiner -
Dortmund, Germany0
Answers
Dortmund, Germany
look at the decision tree. Maybe you left an attribute in the data that correlates so strongly with the label, but wouldn't be available for future data.
Is the tree complex? Are the decisions obvious?
You can put breakpoints on various parts of the process (I'd try with the Decision Tree and Performance) to look at the different validation steps.
Regards,
Balázs
If this happens again, look at the stepwise execution results. If you get a very simple tree, or unbelievable performance results in different executions, the breakpoints help you identify the problem.
Sometimes multiple attributes together correlate with the result but not individually. Decision Tree might be better at catching some of these situations.
Regards,
Balázs
Thats not true. Especially a NB algorithm can be confused very quickly by the other 'noise' attributes. This is not true for a Decision Tree.
Dortmund, Germany