The Altair Community is migrating to a new platform to provide a better experience for you. In preparation for the migration, the Altair Community is on read-only mode from October 28 - November 6, 2024. Technical support via cases will continue to work as is. For any urgent requests from Students/Faculty members, please submit the form linked here
how many trees and datasets are used to optimize random forest?
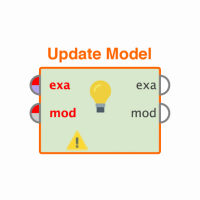

in Help
I'm making predictions that produce fast, medium, and slow predictions. I used 100 trees and around 1000 data training. but always returns fast prediction.
Tagged:
0
Best Answer
-
BalazsBarany Administrator, Moderator, Employee-RapidMiner, RapidMiner Certified Analyst, RapidMiner Certified Expert Posts: 955
Unicorn
Hi!
Do I understand correctly that you're doing classification and your classes are fast, medium and slow?
Sometimes datasets are not suitable for a particular machine learning algorithm, or its default parameters. Sometimes they are imbalanced and then the "best" approach for a machine learning algorithm is to predict the majority class.
Take a look at your data. Is fast overrepresented by a large margin? If it is, can you downsample the class?
Do decision trees, naive bayes, k-NN give you the same result or are they better able to cope with the data?
There are videos in the RapidMiner Academy for topics like sampling and validation that could help you.
Regards,
Balázs1
Answers
i have tried with those and still give the same result
the videos here explain how you sample or weight examples for a more balanced dataset:
https://academy.rapidminer.com/catalog?query=balance
Your data are massively imbalanced. You could also try other approaches like putting together medium+slow into one class (and then possibly a second model for deciding between those), attribute generation (finding some connections between variables that the models don't find, something like area = length * width) and so on.
Regards,
Balázs